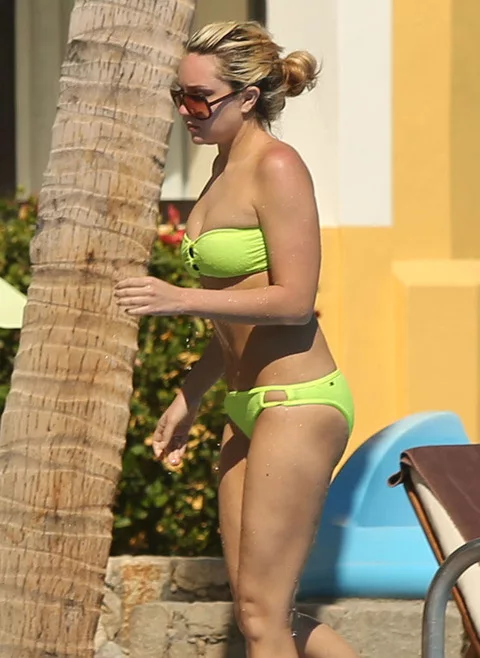
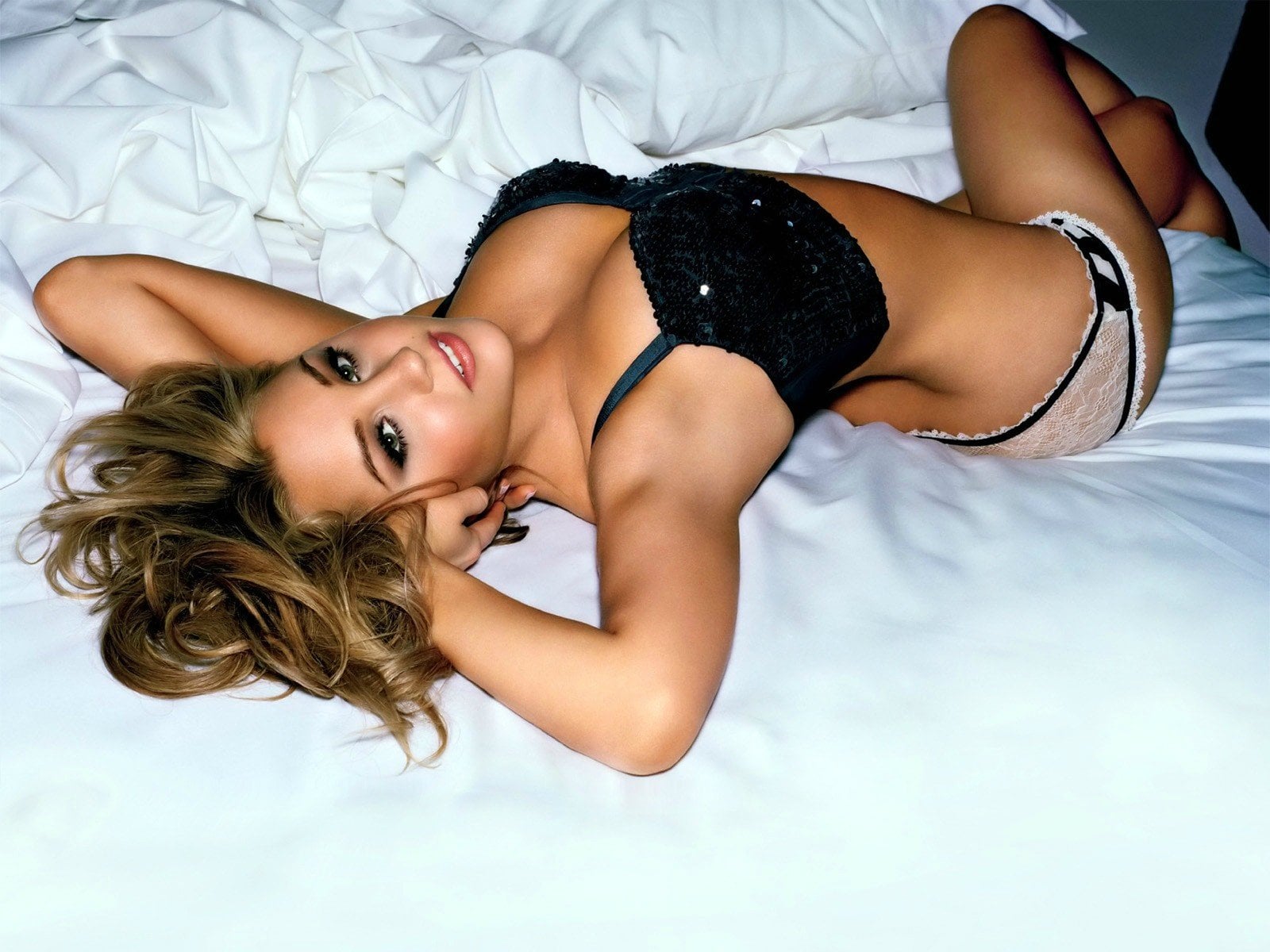
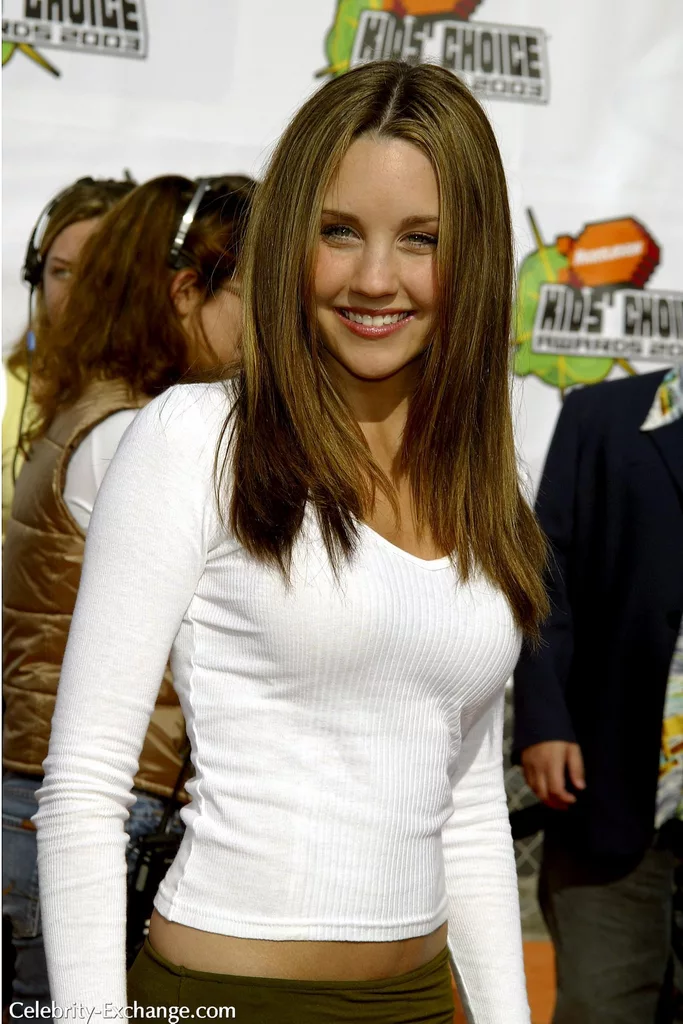
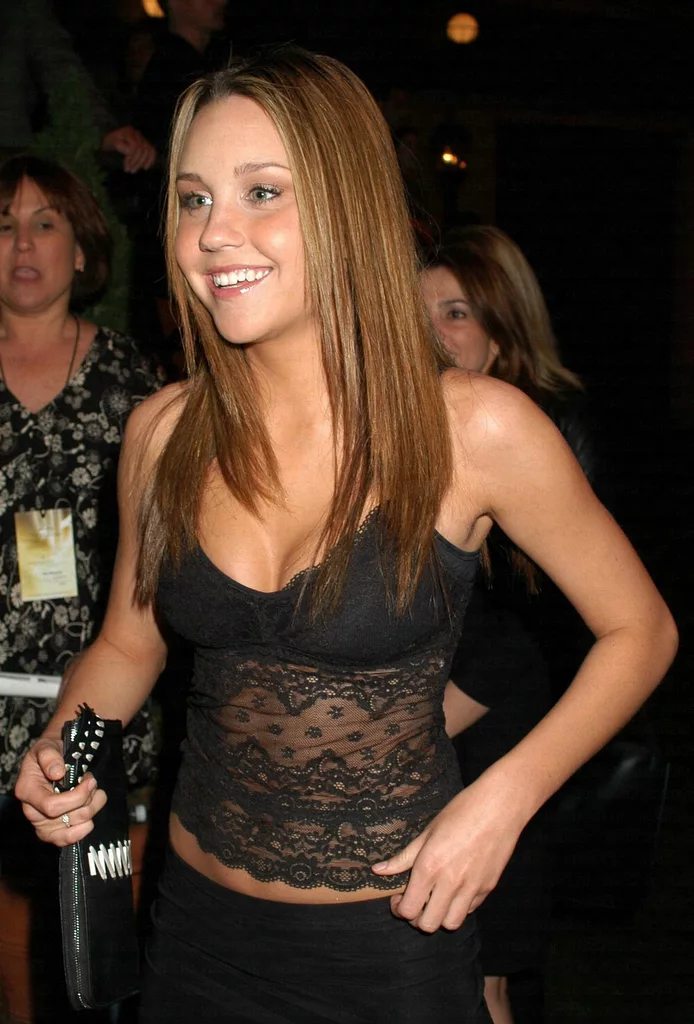

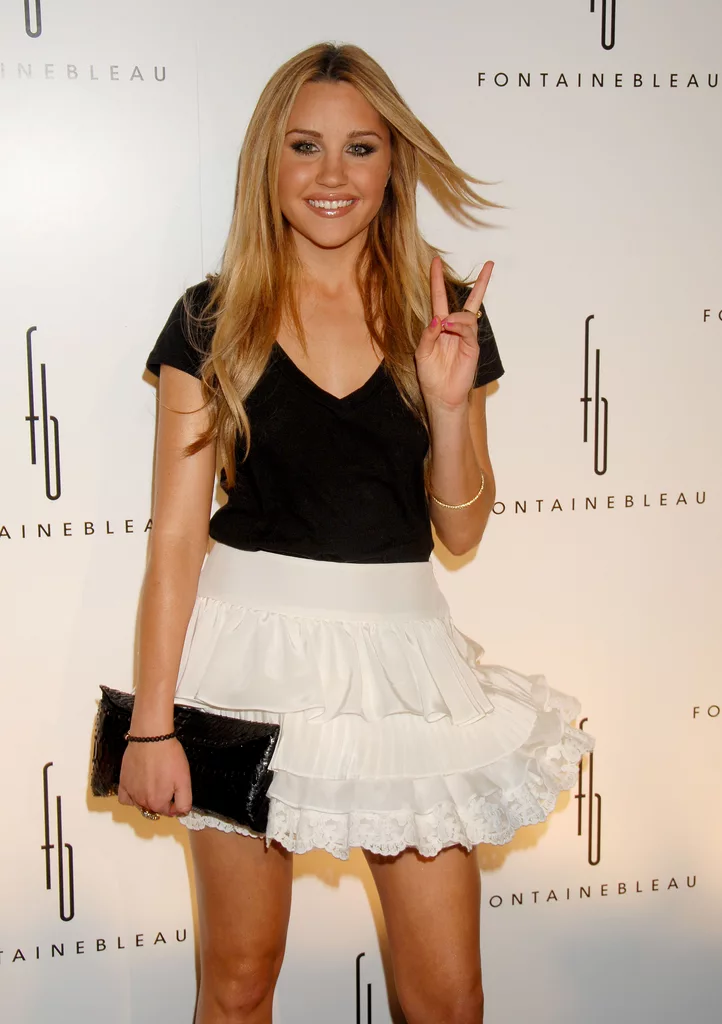
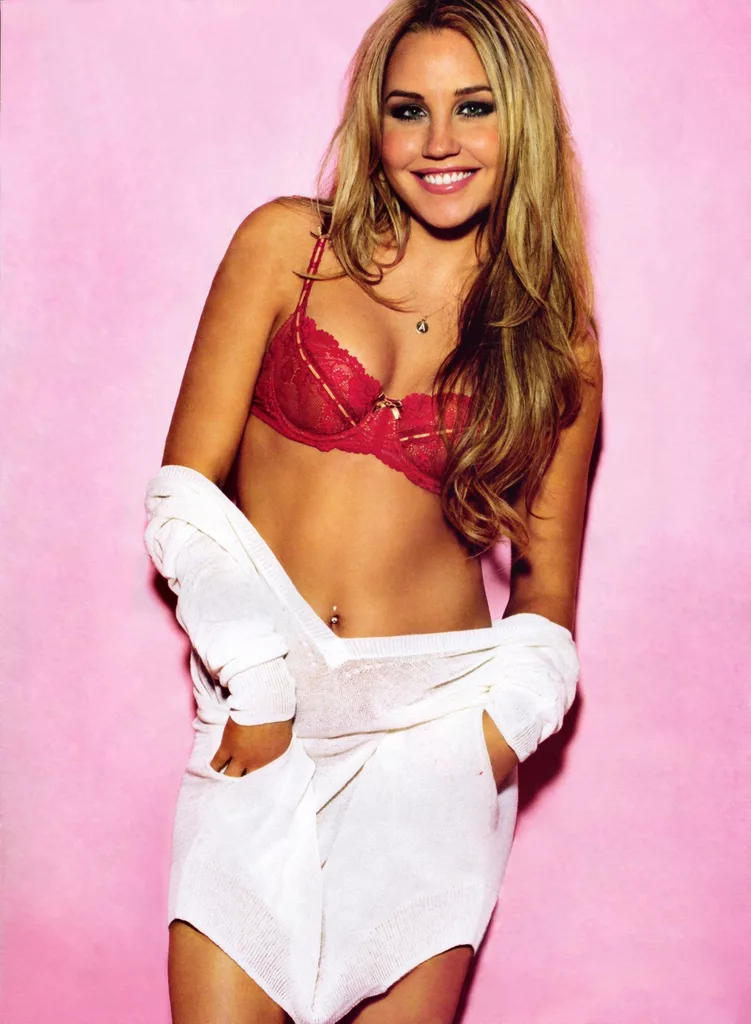
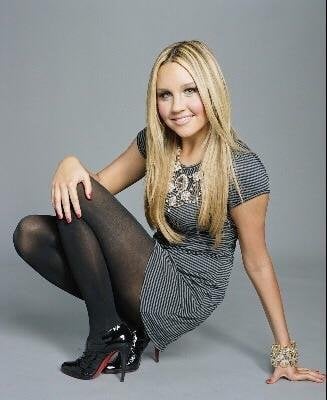
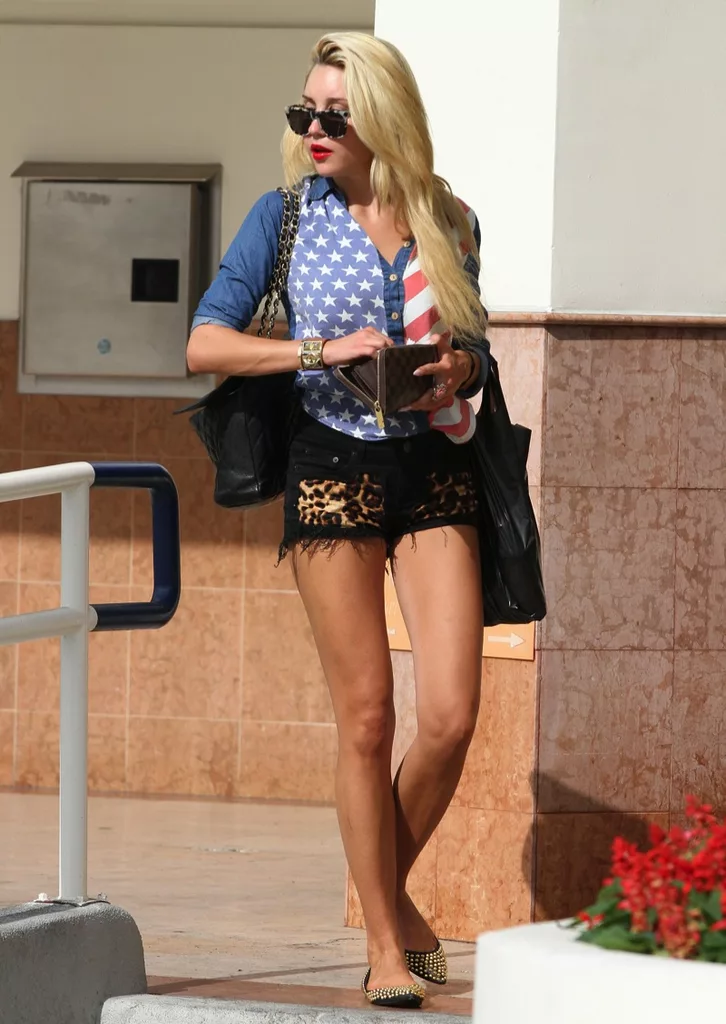
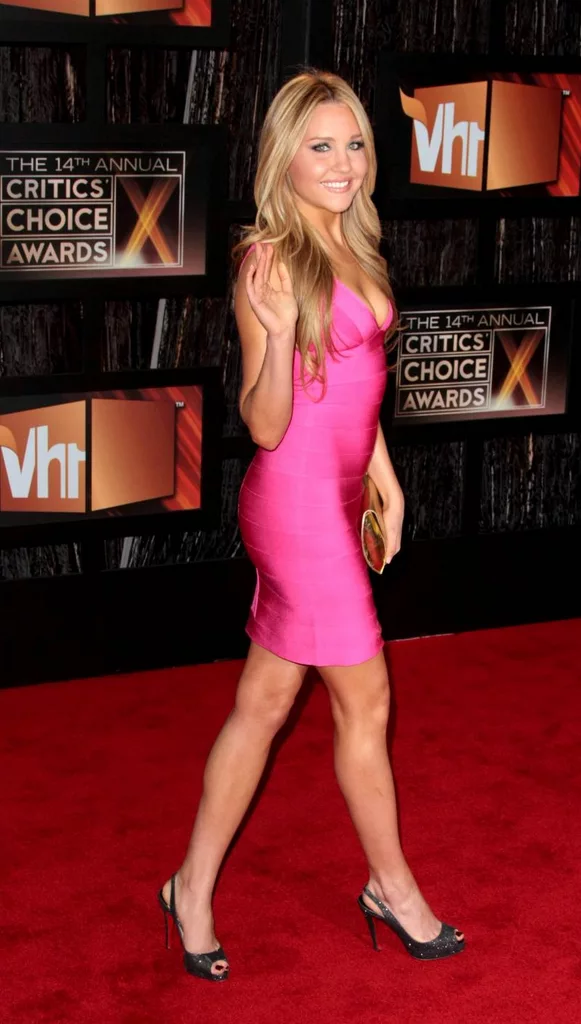
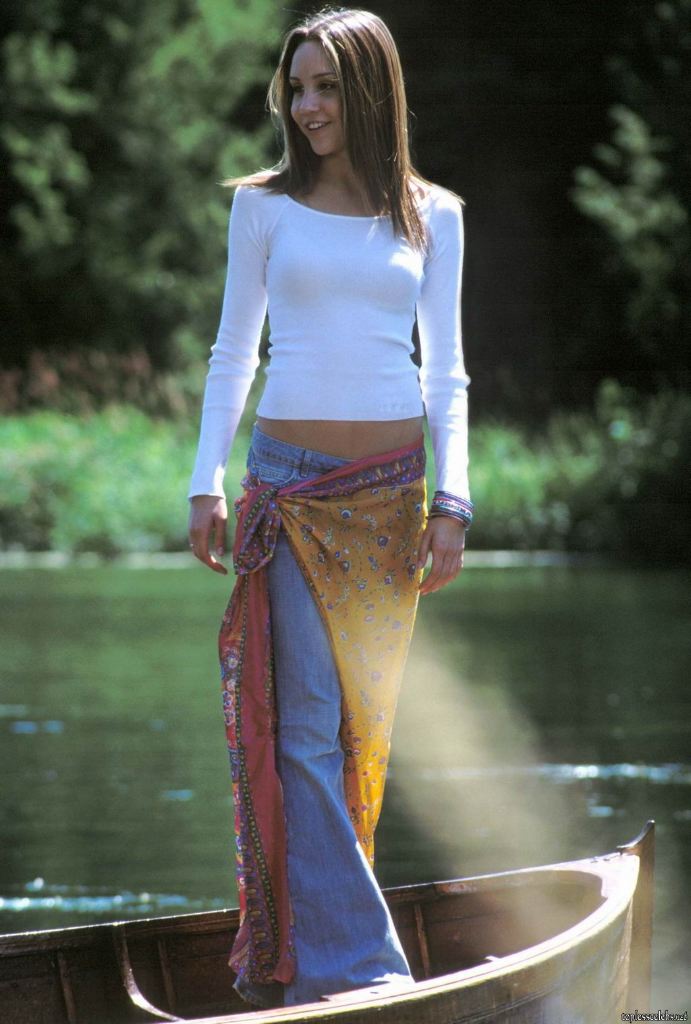
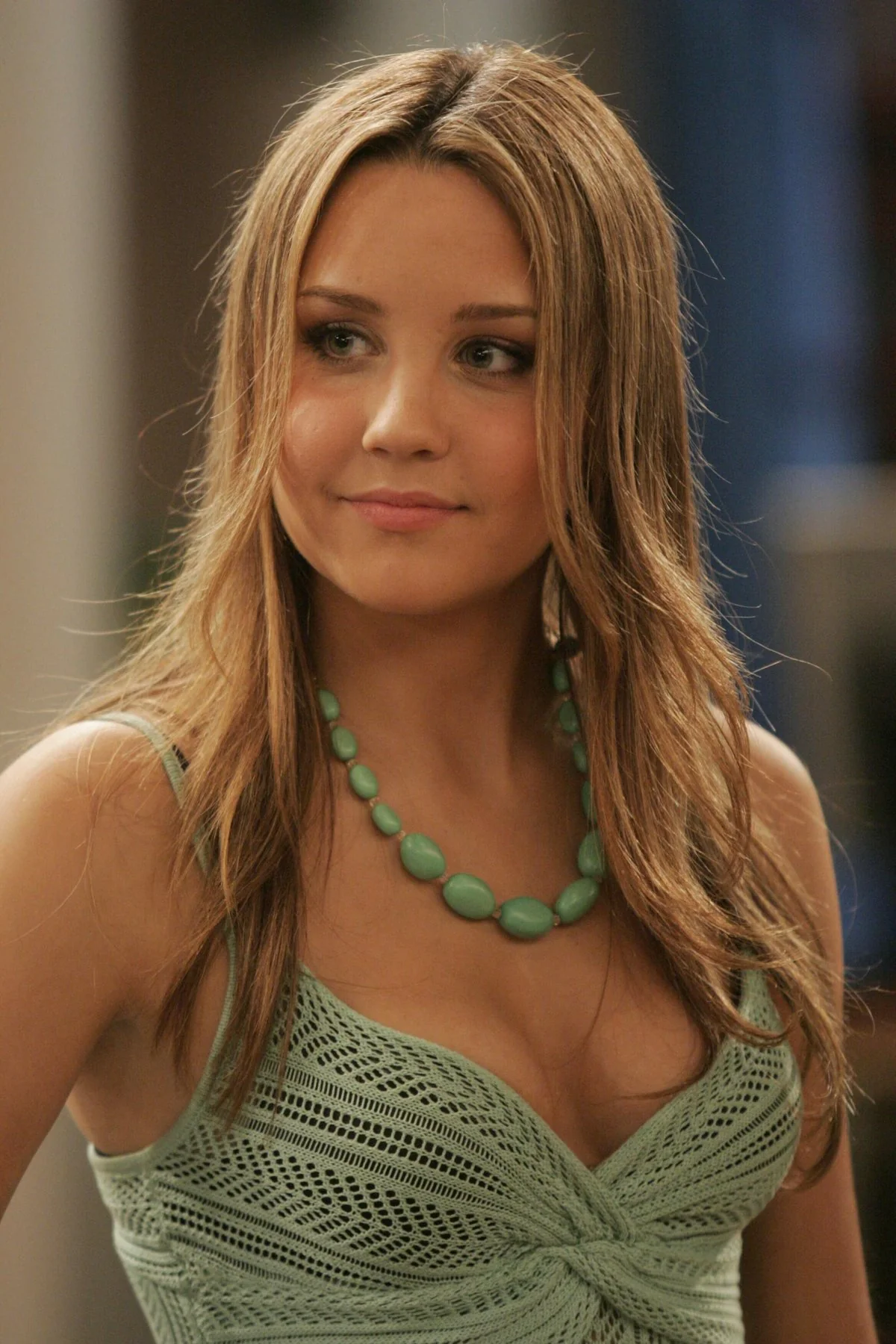
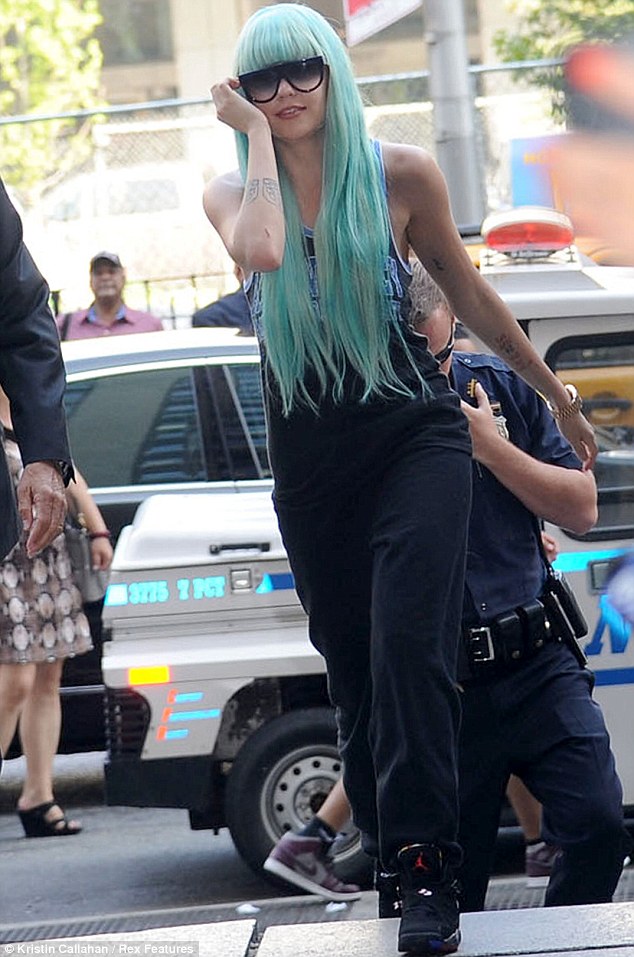
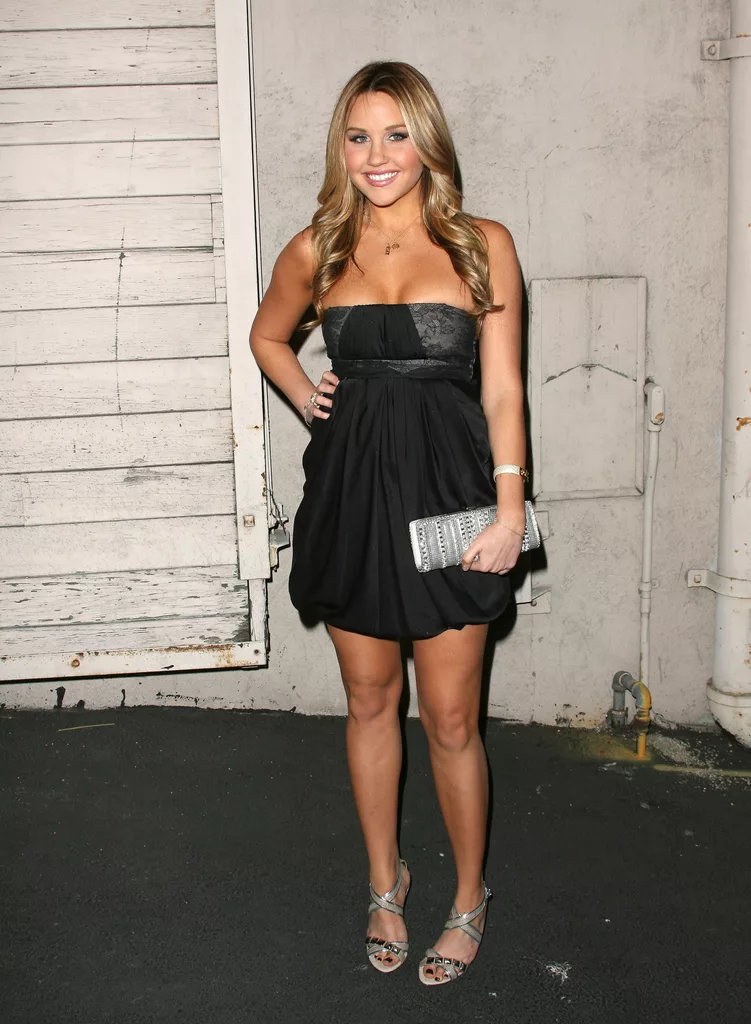
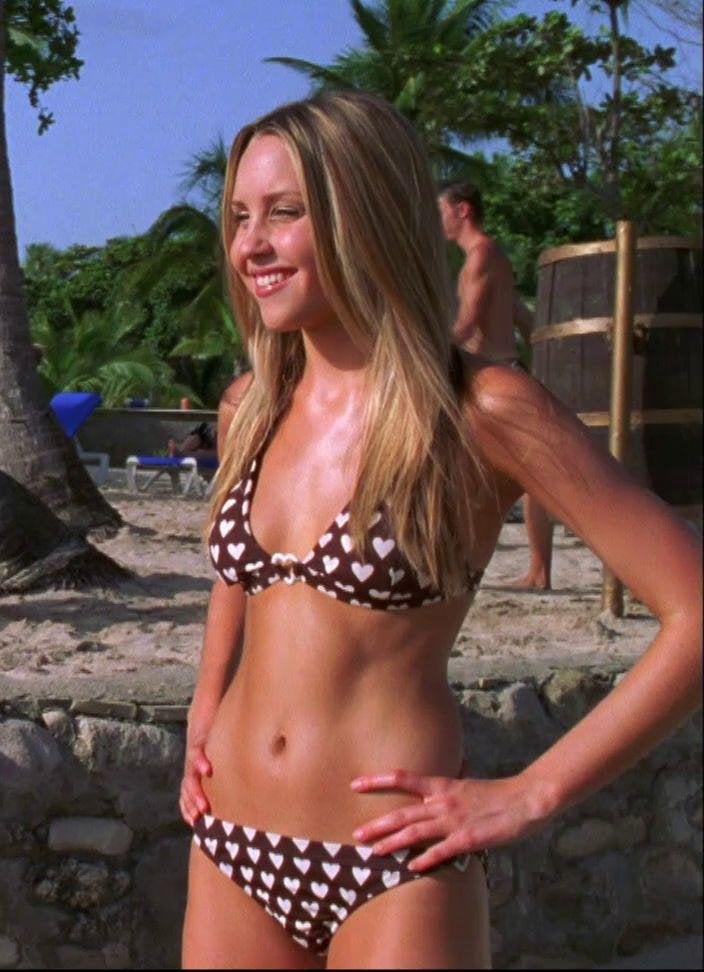
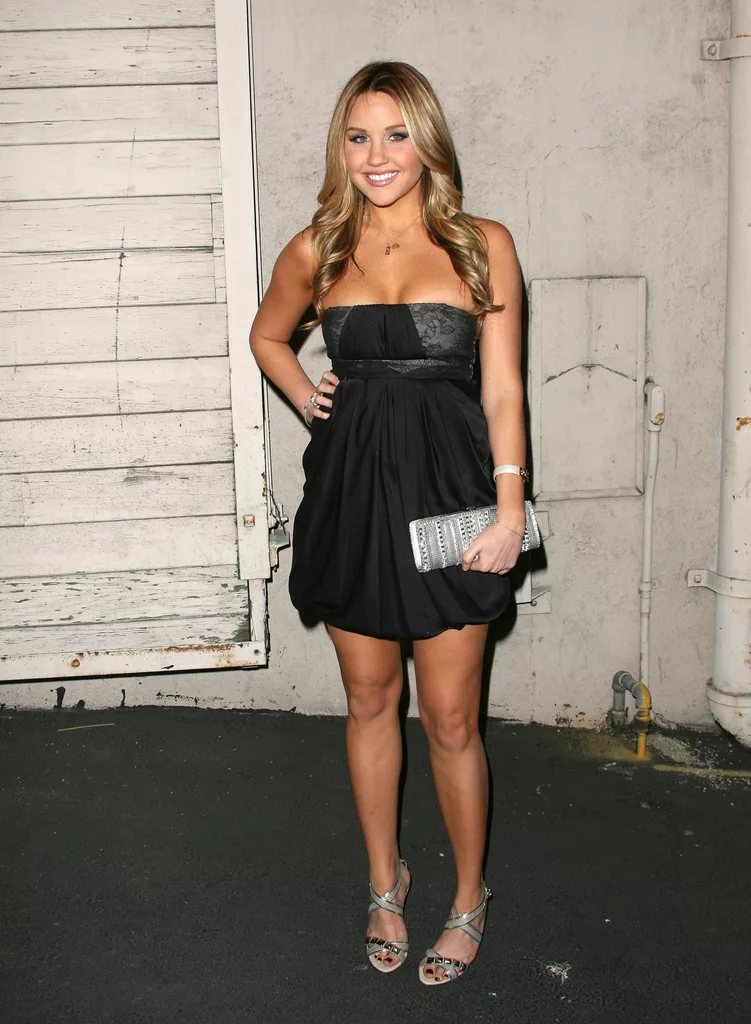

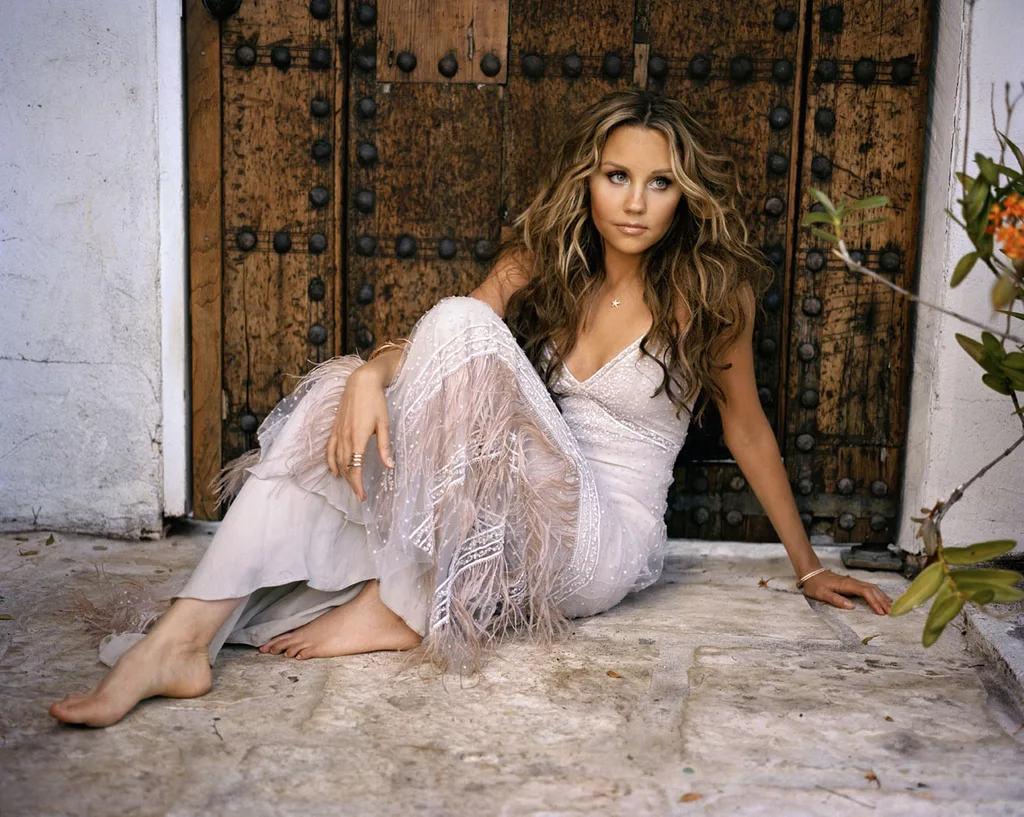
Data is the lifeblood of modern business, driving decisions and shaping strategies. But with vast amounts of data flowing in from various sources, ensuring its integrity and usability becomes a challenge. Two concepts often discussed in this realm are data observability and data quality. Though they may seem similar on the surface, understanding their nuances can make all the difference for organizations looking to leverage their data effectively.
As companies increasingly rely on analytics to inform decision-making, distinguishing between these two critical aspects is essential. Are you prioritizing how well you can monitor your data’s health? Or are you focusing more on making sure that it’s accurate and reliable? Let’s dive into what makes each concept unique and discover how they contribute to a successful data strategy.
Understanding Data Observability and Data Quality
Data observability refers to the ability to monitor and understand data flows in real-time. It involves tracking metrics that provide insights into the health, lineage, and performance of your data systems. By utilizing monitoring tools, organizations can detect anomalies and pinpoint issues quickly.
On the other hand, data quality focuses on ensuring that the information being collected is accurate, complete, and relevant. This encompasses various dimensions such as consistency, validity, and timeliness. High-quality data drives better decision-making across all levels of an organization.
While both concepts are vital for effective data management, they address different aspects of handling information. Data observability emphasizes visibility into processes while data quality ensures reliability in outcomes. Understanding these distinctions lays a strong foundation for any strategic approach toward data governance.
Key Differences Between Data Observability and Data Quality
Data observability and data quality often seem interchangeable, yet they serve distinct purposes in the realm of analytics.
Data observability focuses on monitoring and understanding data flows throughout an organization. It provides visibility into how data moves, transforms, and is consumed. This means identifying anomalies in real-time, ensuring that insights are based on accurate information.
On the other hand, data quality refers to the accuracy, completeness, consistency, and reliability of the data itself. It’s about maintaining high standards for datasets to ensure effective decision-making.
While both concepts aim to enhance business intelligence efforts, their approaches differ significantly. Observability helps detect issues swiftly as they arise; quality management ensures long-term integrity of your datasets. Each plays a critical role in achieving robust analytics solutions but tackles different aspects of data governance.
Importance of Data Observability and Data Quality in Business
Data observability and data quality are crucial for businesses navigating today’s digital landscape. With an overwhelming amount of data generated every day, organizations must ensure they understand their data’s behavior and performance.
When companies invest in data observability, they gain real-time insights into their systems. This enables them to detect anomalies or issues swiftly, reducing downtime and ensuring smooth operations.
On the other hand, maintaining high data quality ensures that decisions are based on accurate and reliable information. Poor-quality data can lead to misguided strategies and lost opportunities.
Together, these concepts empower businesses to enhance their customer experiences while driving analytics initiatives forward. By focusing on both aspects, companies can build trust in their data-driven decision-making processes.
Best Practices for Maintaining Data Observability and Data Quality
Establishing clear data governance policies is essential. This sets the foundation for both data observability and quality. It helps define roles, responsibilities, and standards.
Regularly monitoring your data pipelines can catch issues early. Using automated tools to track changes in data flow enhances visibility. Timely alerts on anomalies prevent small problems from escalating.
Implementing robust testing methodologies ensures that data remains reliable. Continuous validation processes help maintain accuracy during integration or transformation stages.
Collaboration across teams fosters a culture of awareness around data practices. Encourage open communication among stakeholders about any discrepancies they encounter.
Investing in staff training is vital. The more knowledgeable your team is about best practices, the better they’ll manage both observability and quality effectively.
Tools and Technologies for Ensuring Data Observability and Data Quality
When it comes to ensuring data observability and quality, a variety of tools are available to help organizations stay on top of their data game.
Platforms like Monte Carlo and Databand provide robust monitoring features. They track data lineage, detect anomalies, and alert teams about potential issues in real time. This proactive approach allows businesses to maintain high-quality datasets effortlessly.
For those focused on analytics, Looker or Tableau can be invaluable. These solutions not only visualize data but also highlight discrepancies that may indicate underlying problems with quality.
Integrating cloud services such as AWS Glue or Google Cloud Dataflow can streamline workflows while enhancing both observability and quality checks. These technologies automate processes, enabling teams to focus on insights rather than troubleshooting.
Choosing the right tools depends largely on your organization’s unique needs and goals, making careful consideration essential in the selection process.
Choosing the Right Approach for Your Business: A Comparison
When considering the right approach for your business regarding data observability and data quality, it’s essential to assess your unique needs.
Data observability focuses on system health and performance. It provides insights into data flows and processing pipelines. If you’re dealing with real-time analytics or complex workflows, prioritizing observability may be key.
On the other hand, if accuracy and reliability of information are paramount—think compliance-heavy industries—data quality takes precedence. Ensuring clean, consistent datasets can enhance decision-making processes.
Evaluate scalability as well. Your choice should align with growth plans while accommodating evolving requirements in both areas.
Budget constraints also matter; some tools might offer robust features but come at a higher cost. Factor in potential ROI when making comparisons.
Aligning these approaches with business objectives will guide you toward the best fit for fostering a data-driven culture.
Conclusion
Data observability and data quality may seem interchangeable at first glance, but understanding their nuances is crucial for any business that relies on data. Both are essential components in creating a robust data strategy.
Data observability focuses on the visibility into your data systems, allowing you to monitor and troubleshoot issues effectively. On the other hand, data quality emphasizes ensuring that the information coming from these systems is accurate, reliable, and relevant.
The importance of both cannot be overstated. High-quality data enhances decision-making processes while effective observability can prevent costly errors before they escalate. Businesses that prioritize these elements often see improved operational efficiency and better insights from their analytics.
Maintaining a strong framework for both concepts involves implementing best practices such as regular monitoring, using automated tools for detection of anomalies, and conducting frequent audits to ensure compliance with established standards.
When it comes to choosing tools or technologies for enhancing your strategies around data observability vs data quality, there are numerous options available in the market today—each offering unique features tailored to specific needs.
Aligning your approach with your specific business objectives will yield the best results. Understanding how each facet contributes uniquely allows organizations not only to fix current issues but also proactively manage future challenges stemming from poor visibility or low-quality data sources. Making informed decisions ensures long-term success in an increasingly intricate digital landscape filled with vast amounts of ever-evolving information.