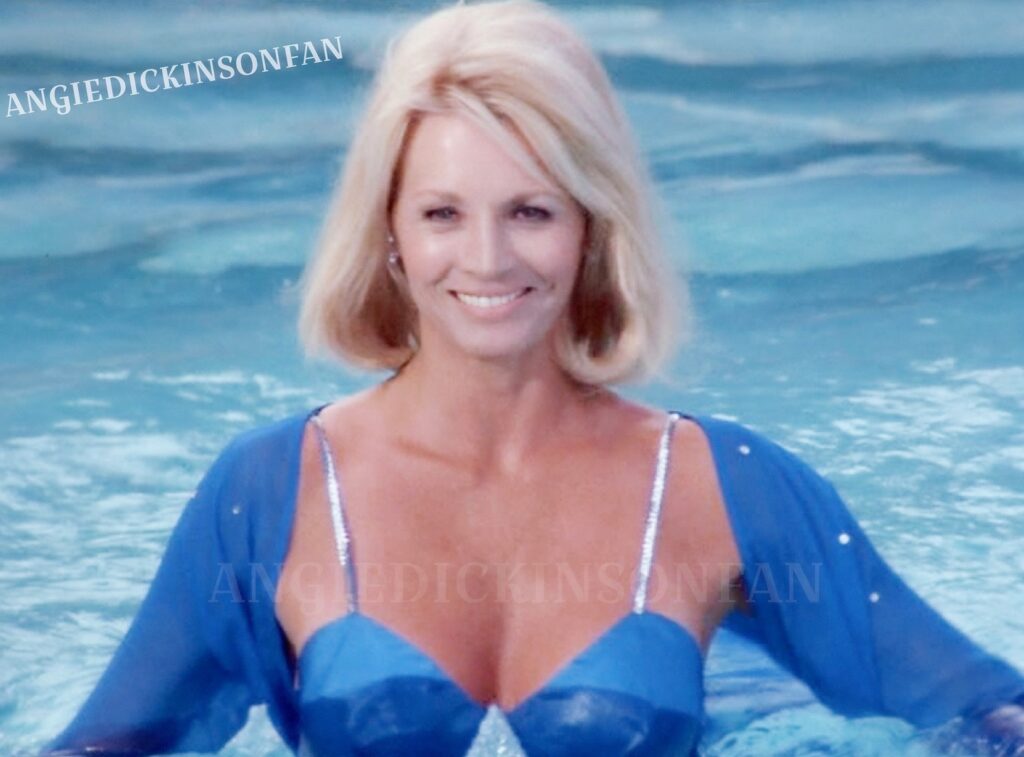
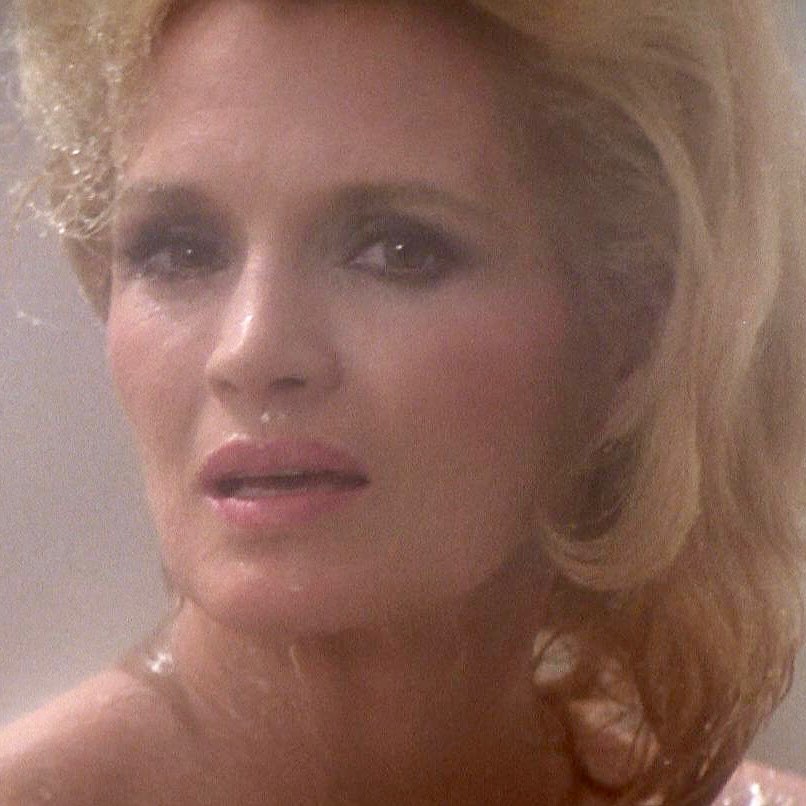
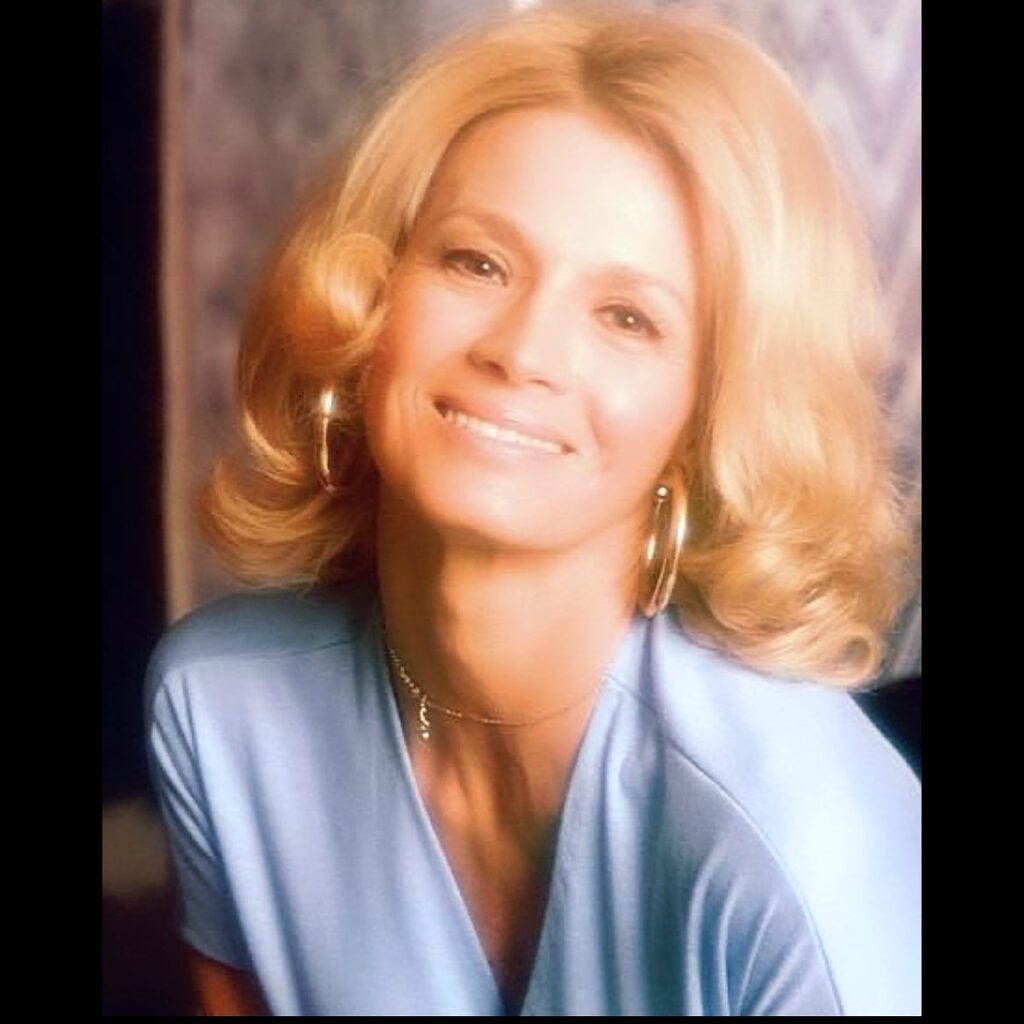

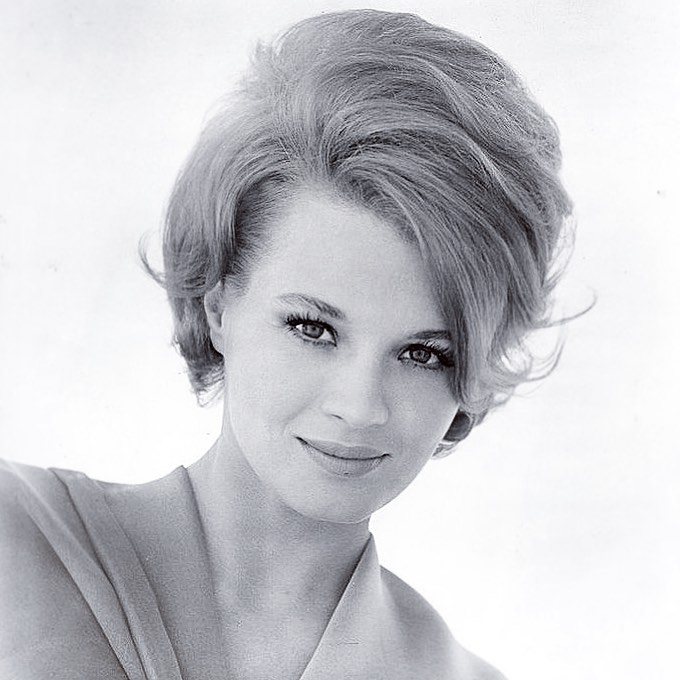
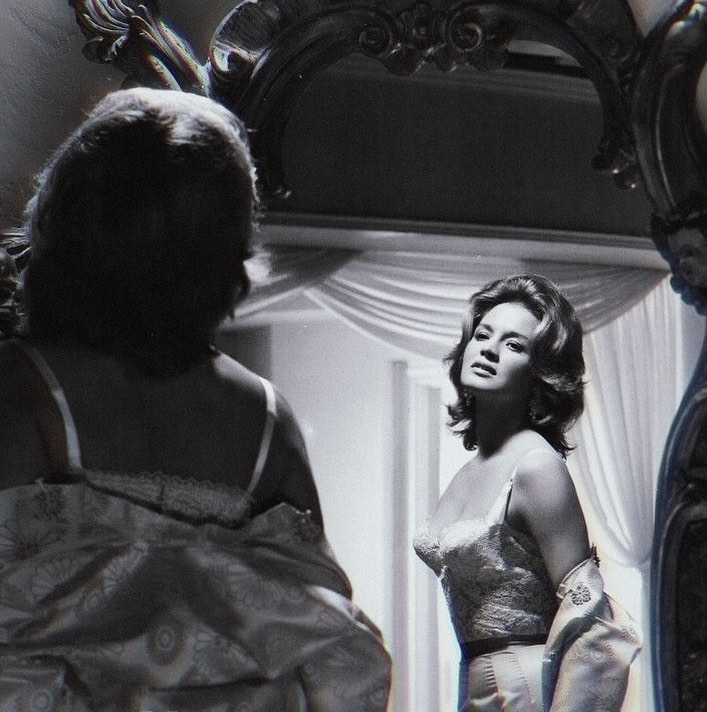
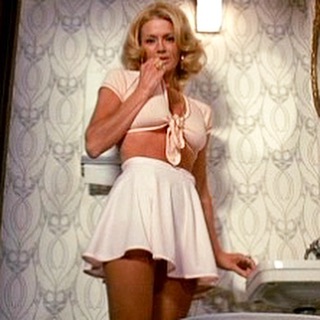
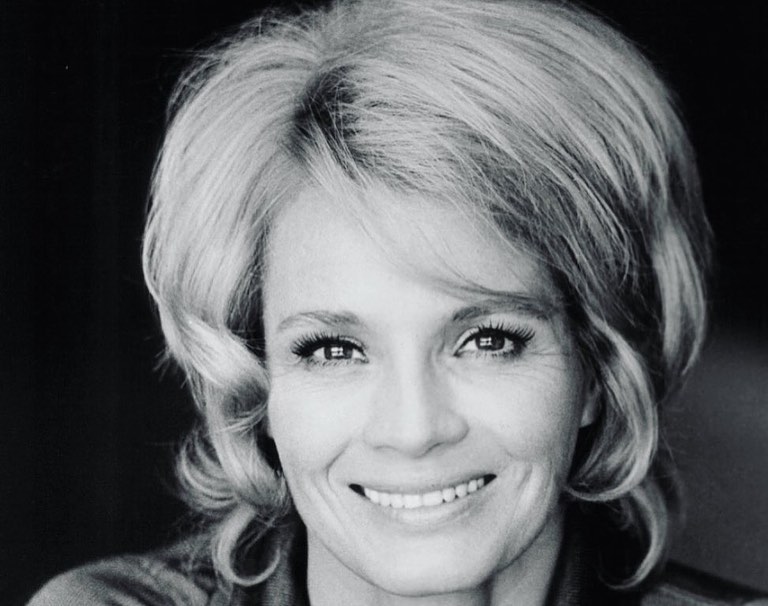
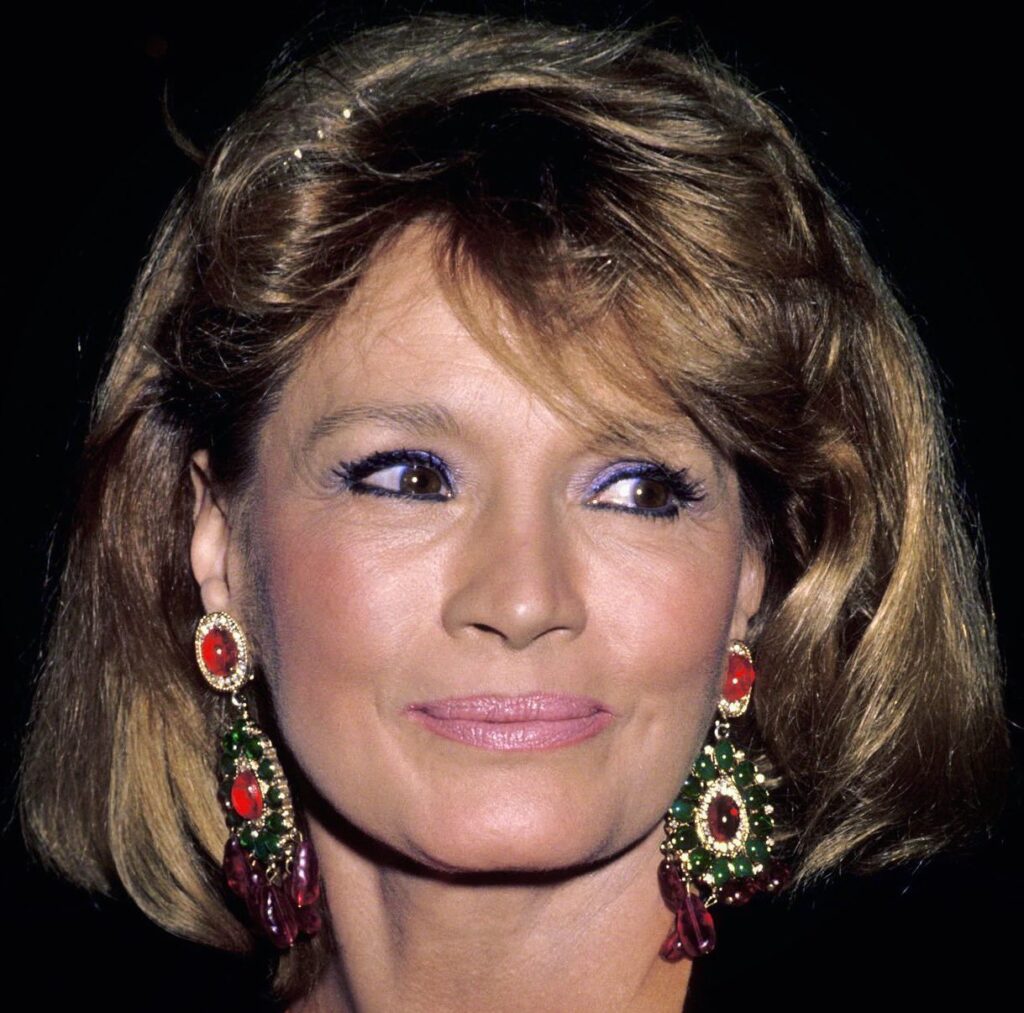
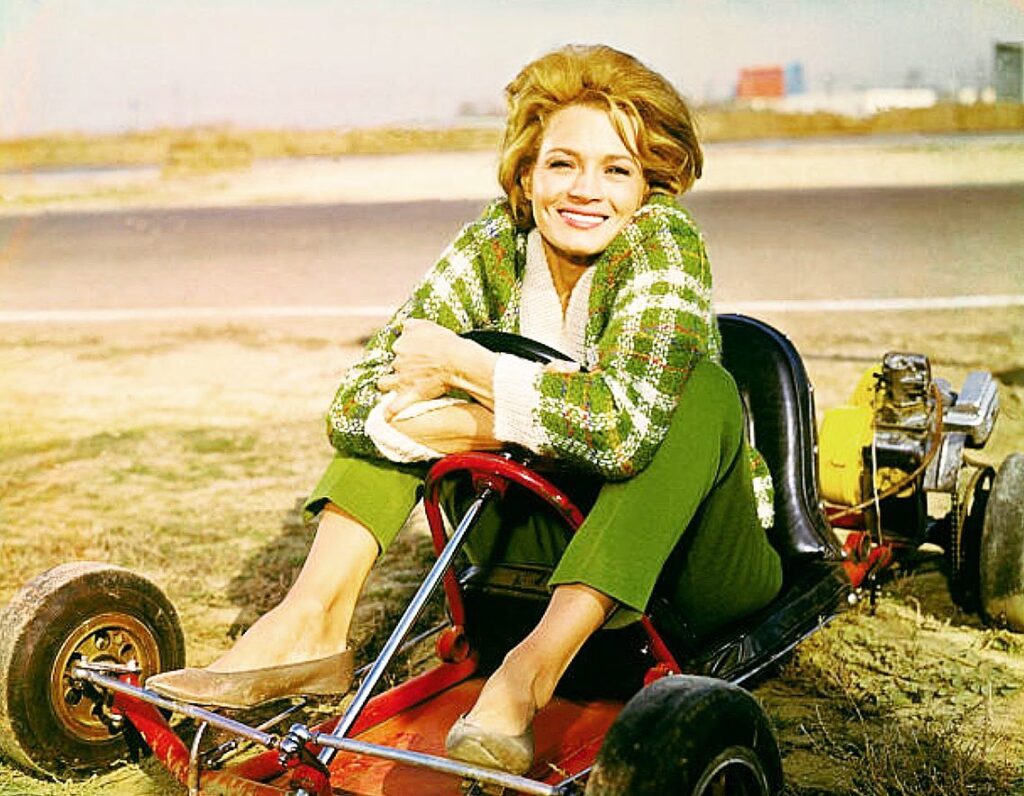
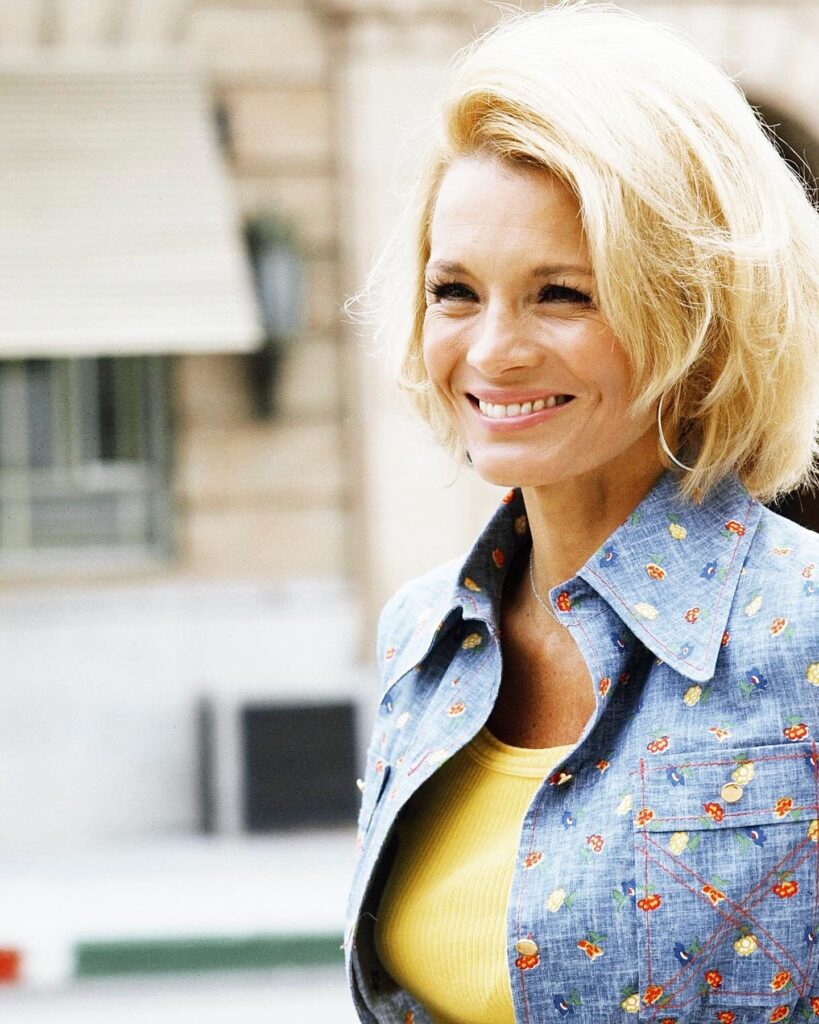
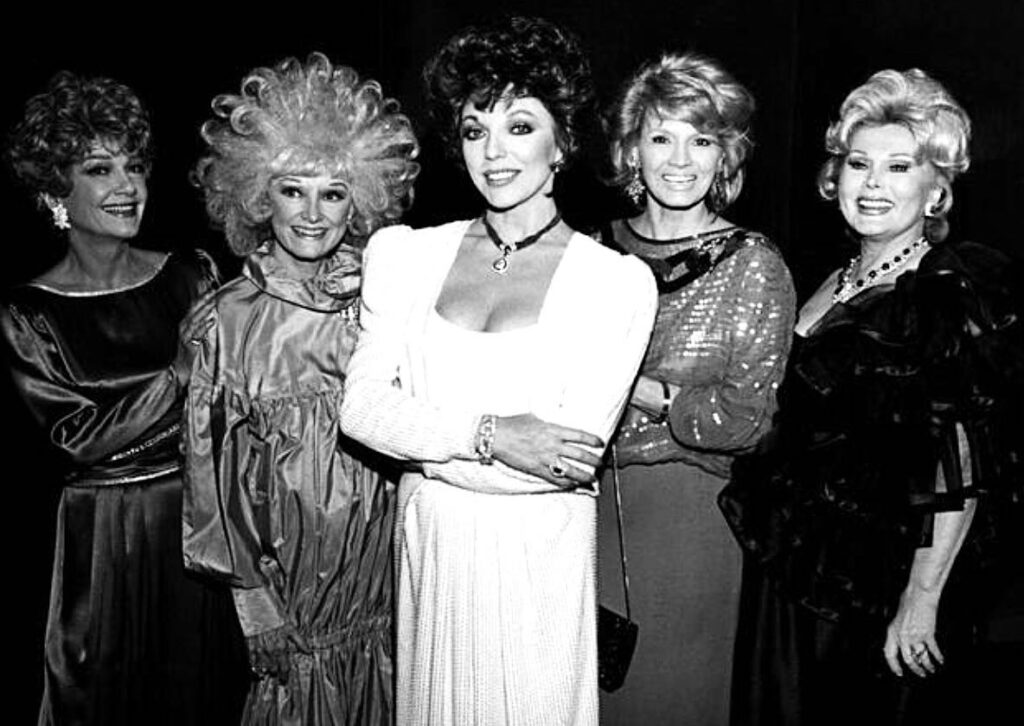
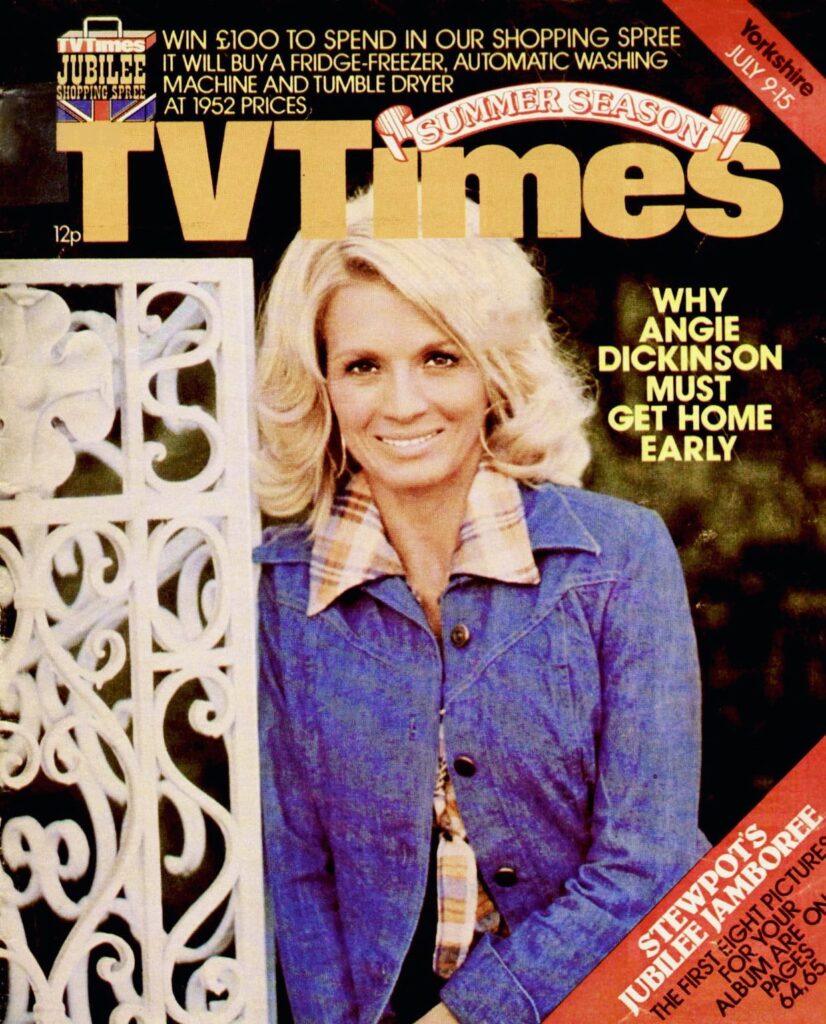
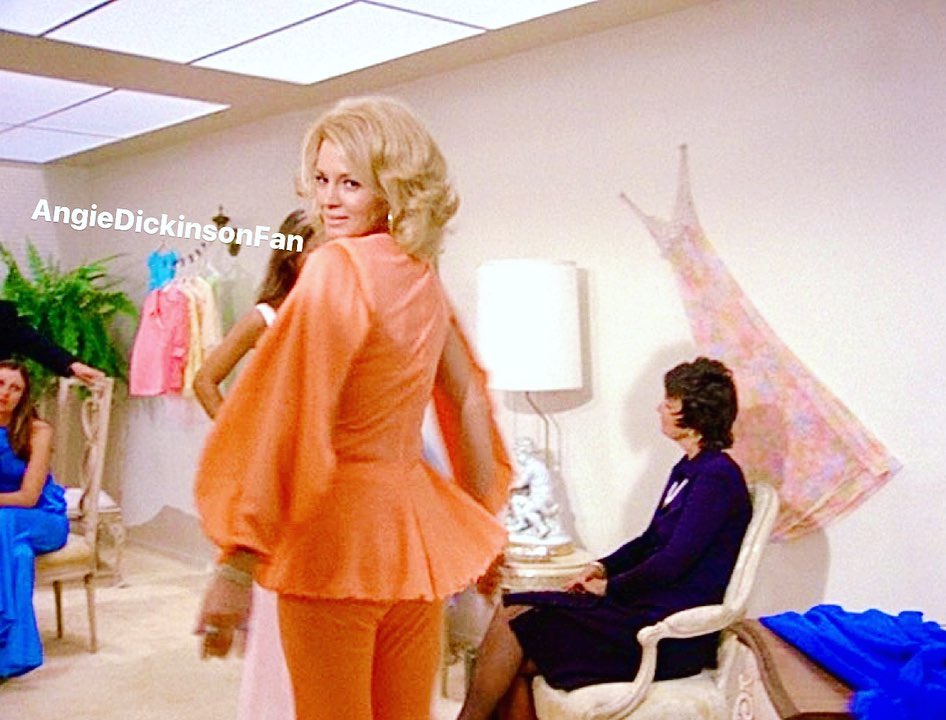
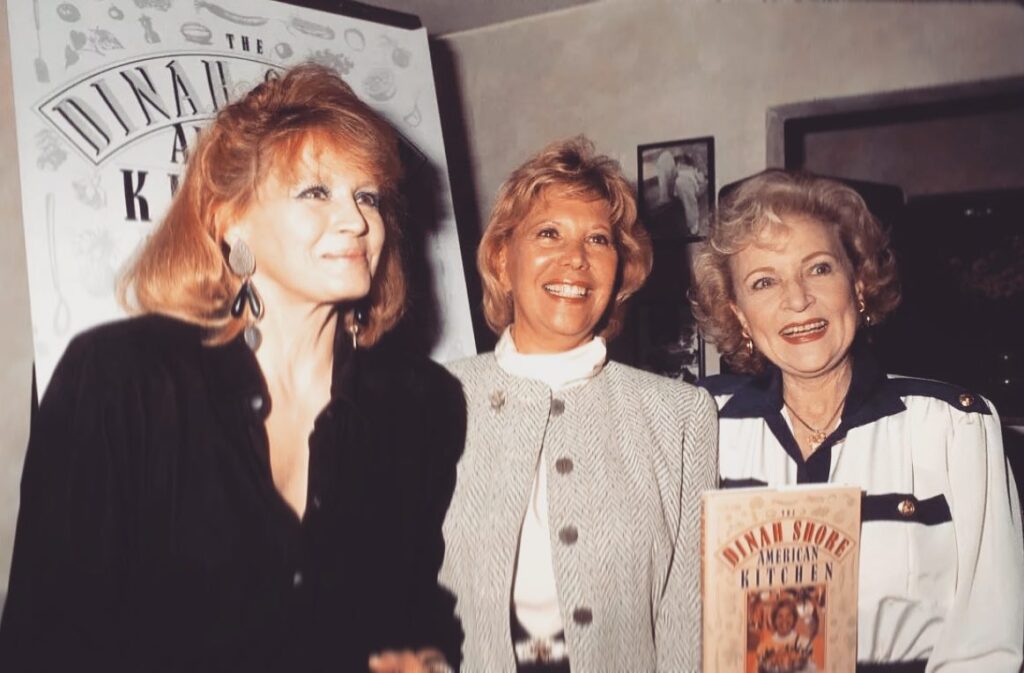
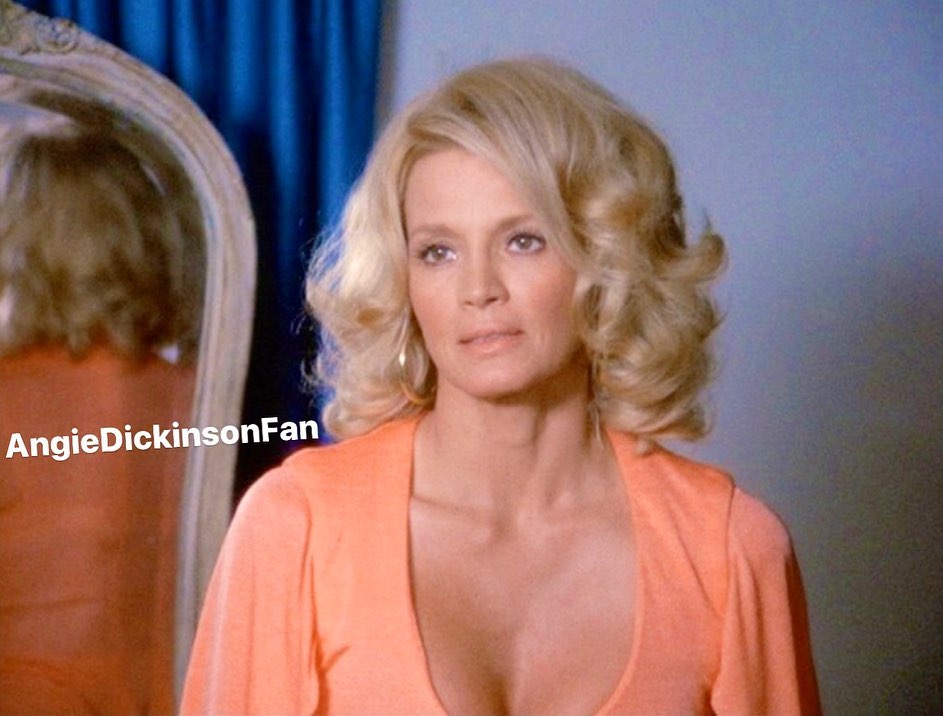
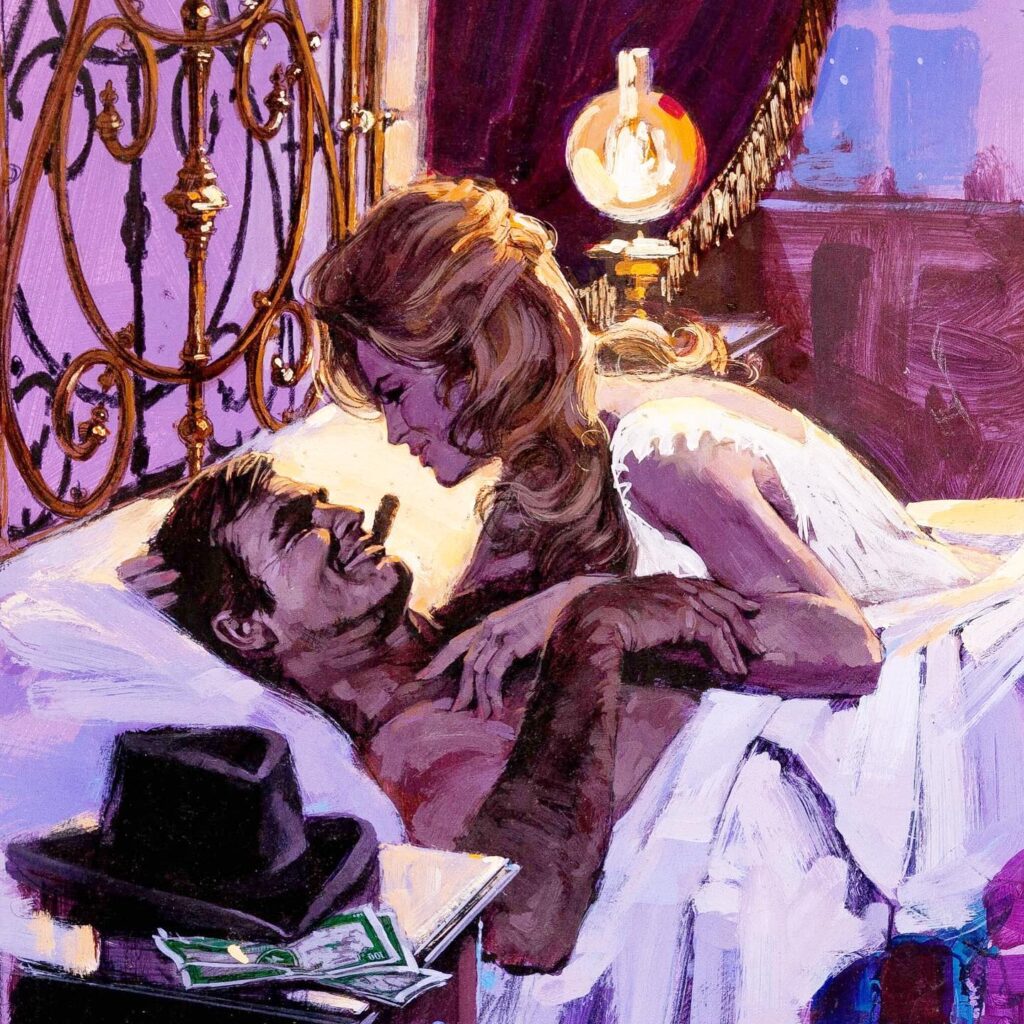
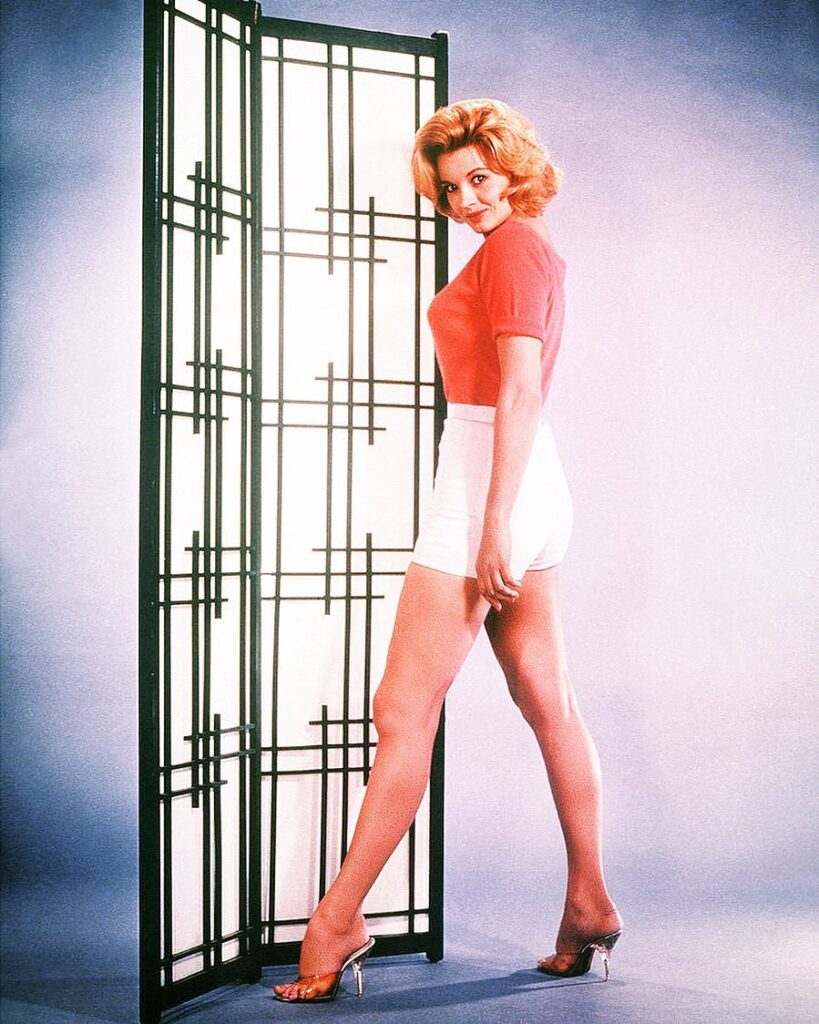
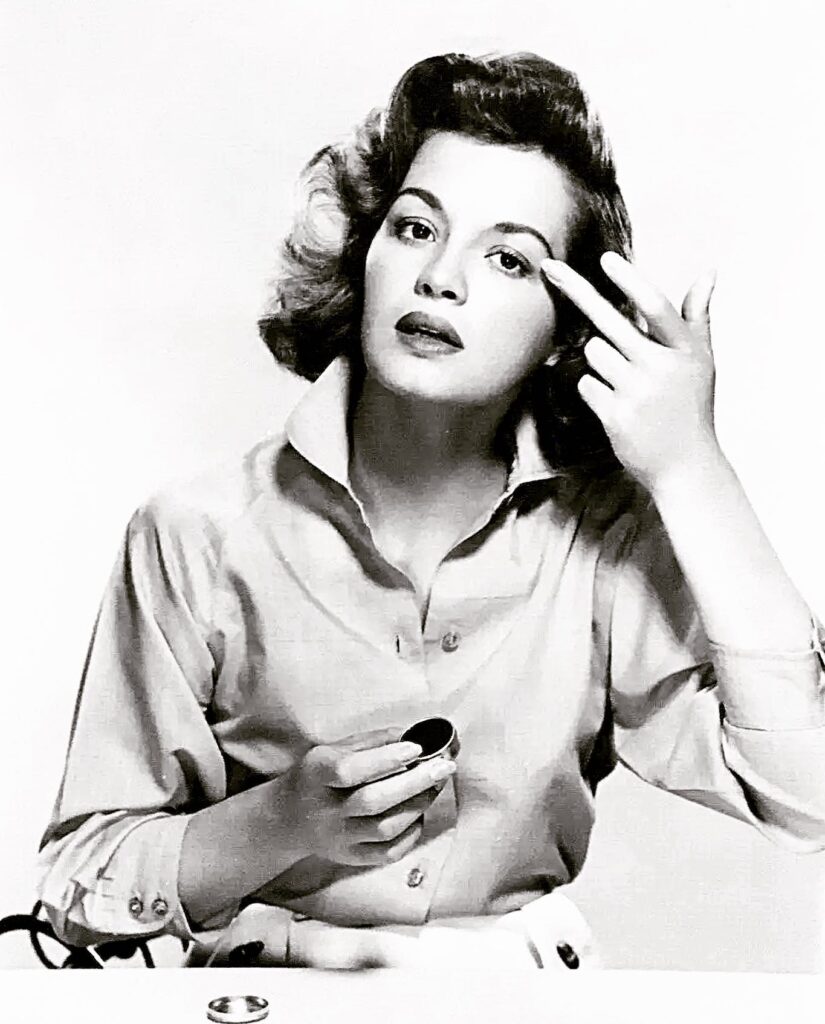
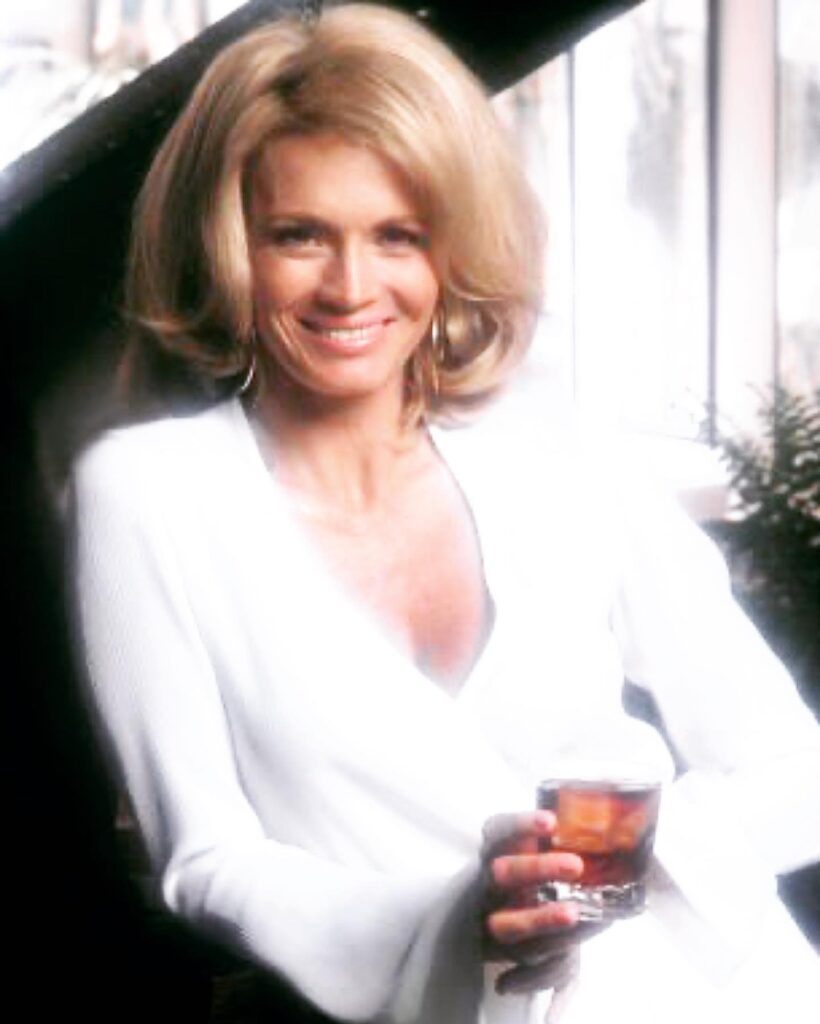
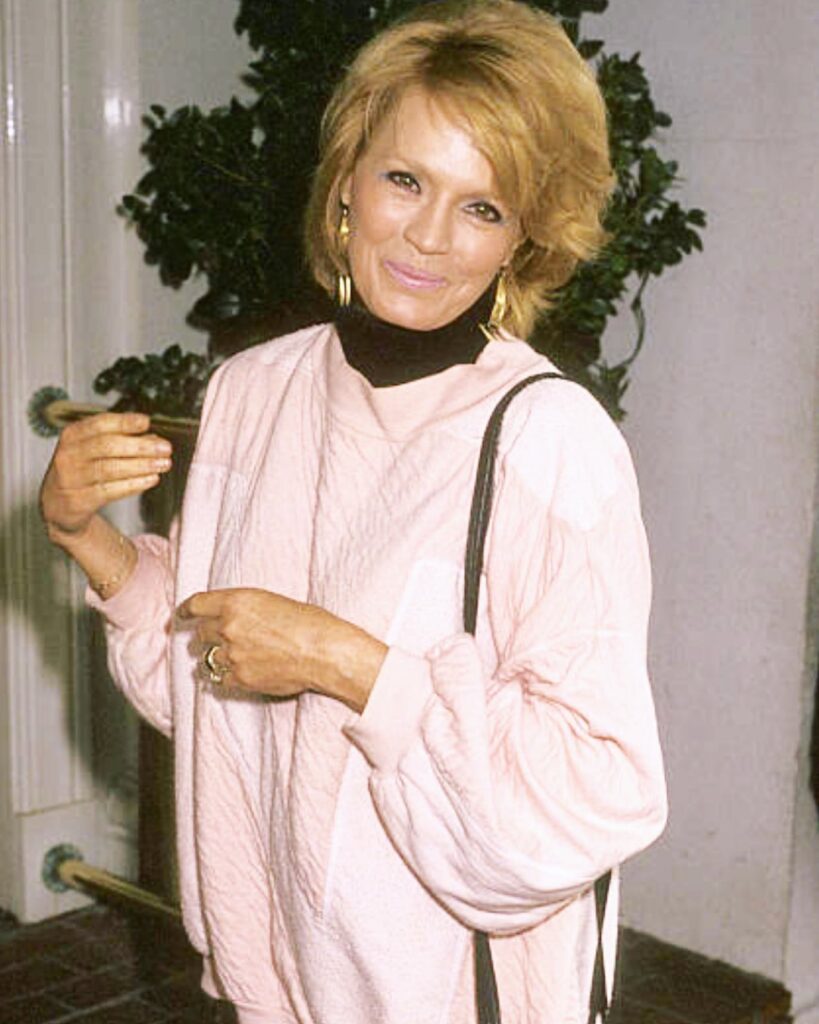
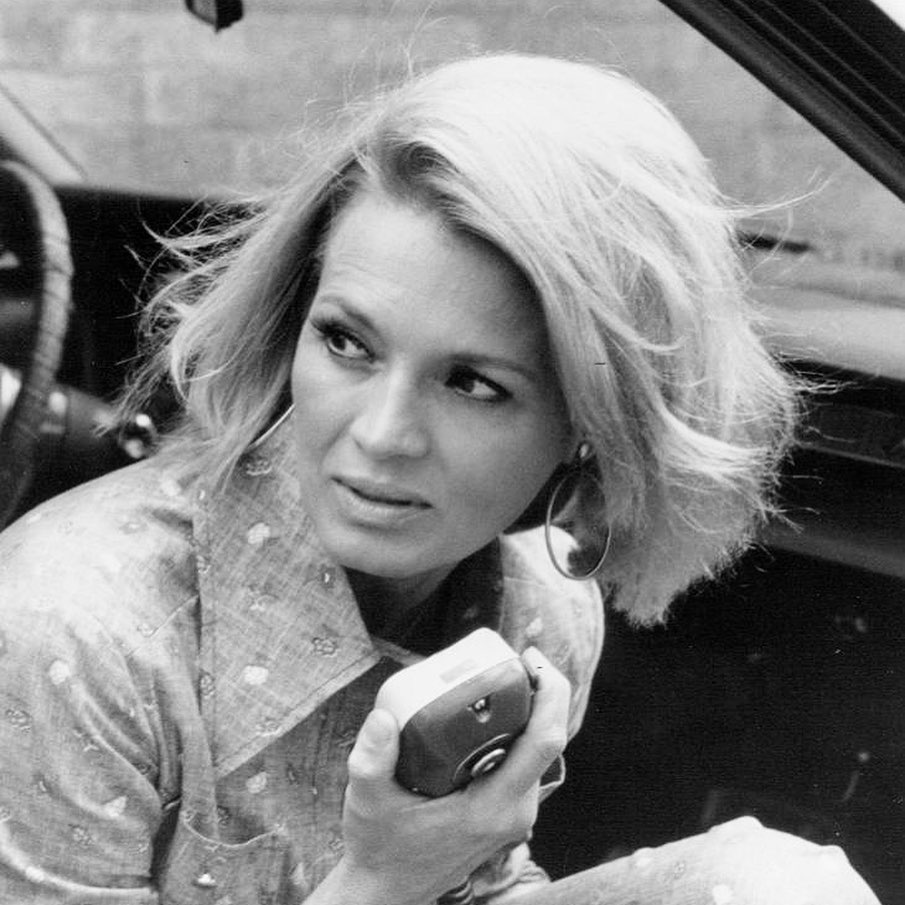
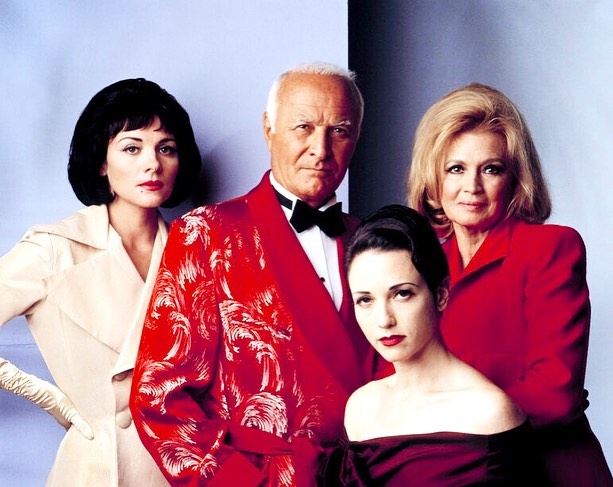
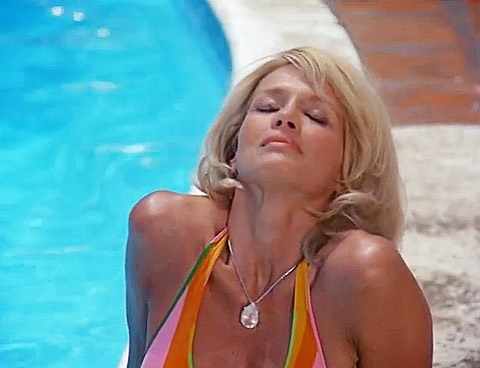
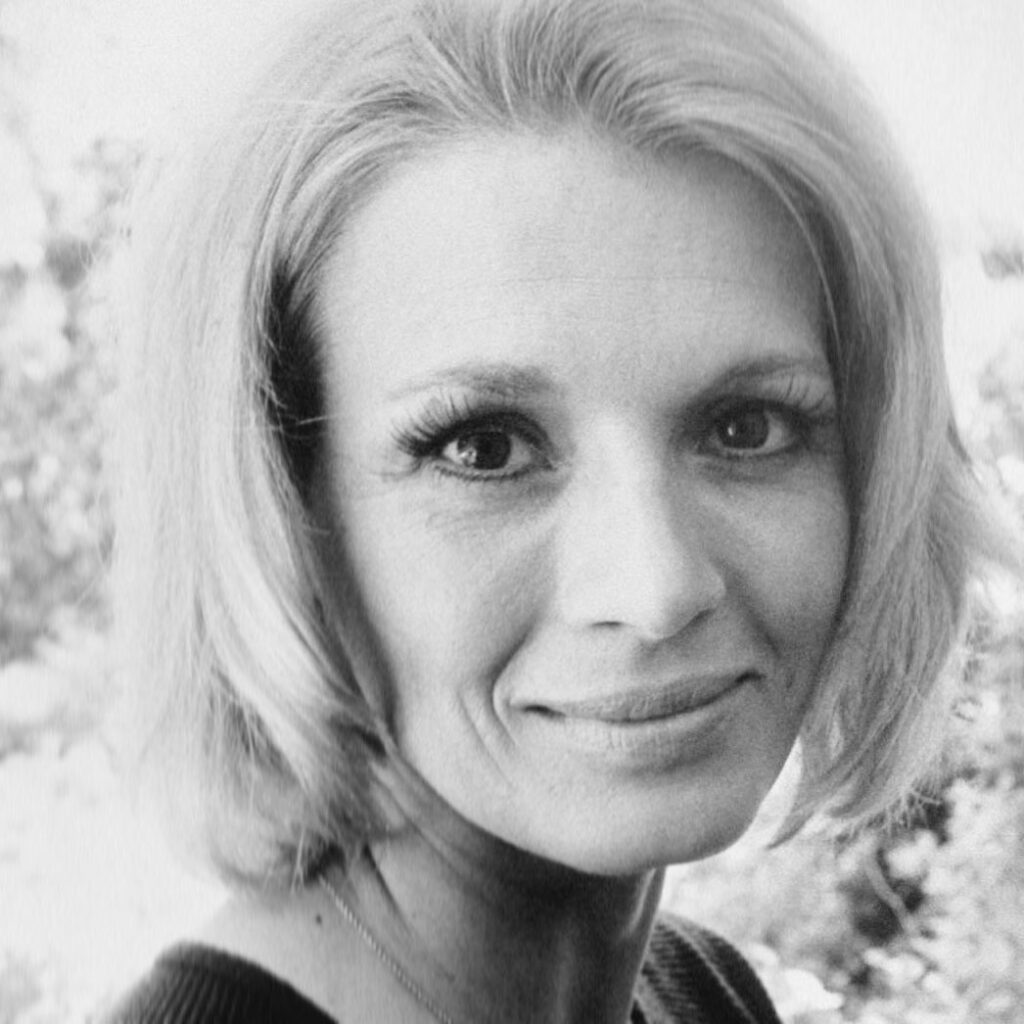
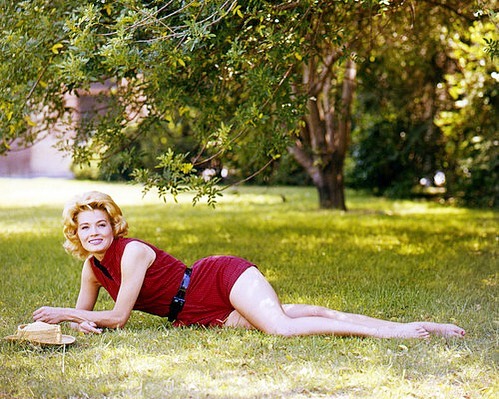
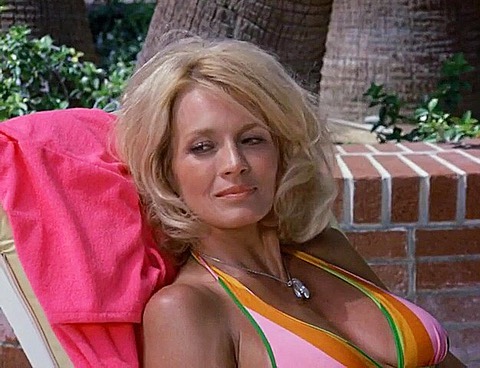
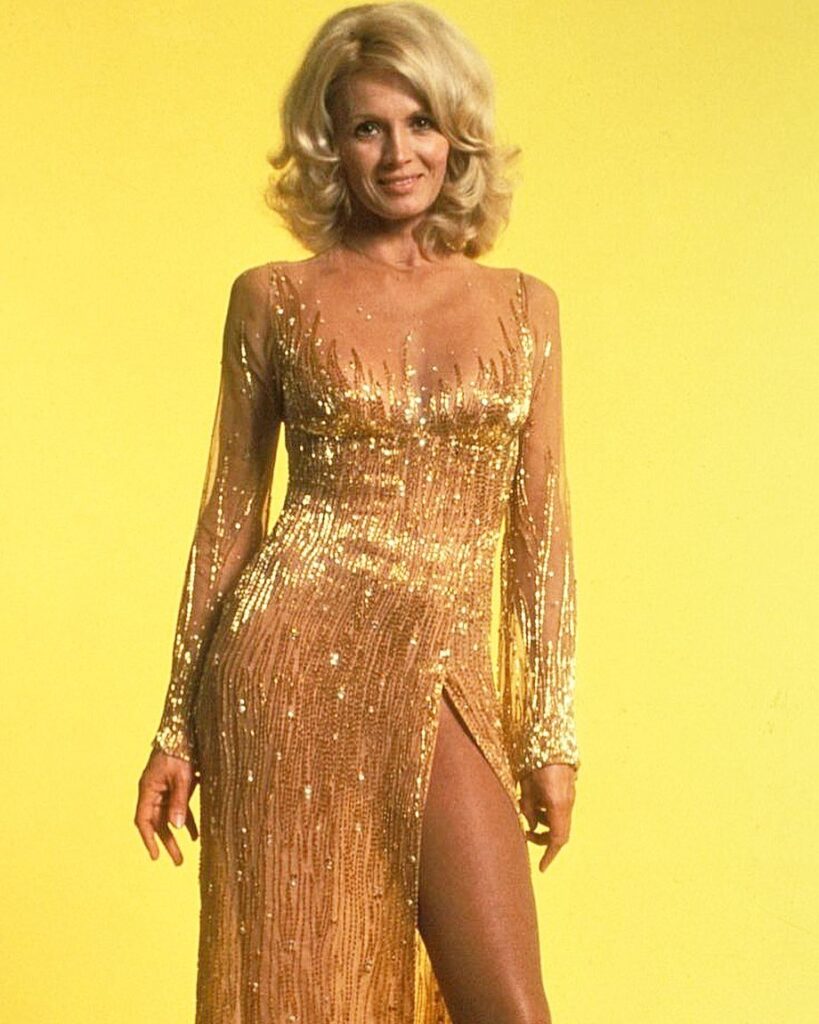
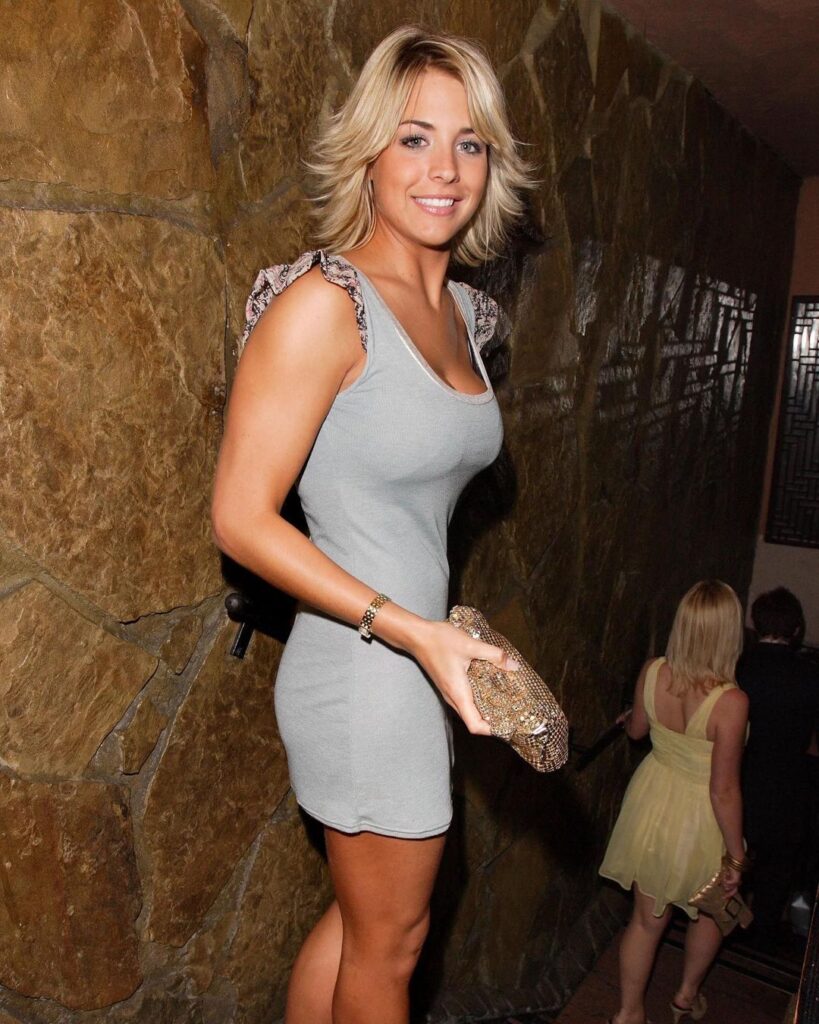
In the competitive world of modern manufacturing, operational efficiency is paramount. Companies are constantly striving to minimize downtime, reduce maintenance costs, and optimize production schedules. Traditional maintenance practices, such as reactive or preventive maintenance, are being gradually replaced by more advanced, data-driven approaches. One such approach is AI-driven predictive maintenance, which leverages the power of artificial intelligence (AI) to forecast potential equipment failures before they occur, leading to significant cost savings, enhanced productivity, and improved equipment reliability.
What is Predictive Maintenance?
Predictive maintenance (PdM) refers to the practice of using data analysis tools and techniques to predict when equipment or machinery is likely to fail. This approach allows manufacturers to perform maintenance only when necessary, rather than following a fixed schedule or waiting for a breakdown. Unlike preventive maintenance, which is based on time intervals or usage cycles, predictive maintenance uses real-time data to assess the condition of machinery and predict the optimal time for intervention.
By integrating AI with predictive maintenance, manufacturers can unlock more precise, accurate, and actionable insights, enabling them to take proactive steps in addressing potential issues before they lead to costly downtime or production delays.
How AI Drives Predictive Maintenance
AI-driven predictive maintenance involves the use of machine learning (ML), deep learning (DL), and data analytics algorithms to analyze data collected from industrial equipment. Here’s how AI enhances predictive maintenance:
- Data Collection and Monitoring Sensors are installed on machinery and equipment to collect real-time data on a variety of operational parameters, such as temperature, vibration, pressure, speed, and more. This data is then sent to an AI system for analysis. AI algorithms continuously monitor this incoming data to detect patterns or anomalies that may indicate impending failure.
- Data Analysis and Pattern Recognition AI-powered predictive maintenance platforms analyze historical and real-time data to identify trends and patterns that are indicative of potential failures. Using machine learning algorithms, these systems can detect even subtle signs of wear and tear or deviations from normal operation that might go unnoticed by human operators.
- Fault Diagnosis and Prediction Once the system identifies abnormal patterns, AI models can predict when and how the equipment might fail. These models use historical data, past failures, and operational conditions to estimate the remaining useful life (RUL) of machinery components, providing valuable insights into when maintenance should be scheduled. The AI system can then generate alerts for maintenance teams to act on these predictions.
- Optimization of Maintenance Schedules Based on predictive insights, AI-driven systems can recommend optimal maintenance schedules, allowing manufacturers to prioritize interventions based on urgency and potential impact. By performing maintenance only when necessary, manufacturers can avoid unnecessary downtime and reduce maintenance costs associated with over-servicing or prematurely replacing parts.
- Continuous Learning and Improvement One of the standout features of AI is its ability to learn and improve over time. As AI systems are exposed to more data, they continuously enhance their predictions, becoming more accurate and reliable. This ongoing learning process helps refine predictive models and ensures that manufacturers can make more informed decisions about when and how to maintain their equipment.
Benefits of AI-Driven Predictive Maintenance
- Reduced Downtime Predictive maintenance helps manufacturers avoid unexpected equipment failures by predicting potential breakdowns before they occur. This leads to fewer unplanned downtimes, which can be costly in terms of lost production and emergency repairs. By addressing issues before they escalate, AI-driven systems ensure that machines run smoothly and without interruptions.
- Cost Savings Traditional preventive maintenance can be costly, as it involves performing routine checks and replacing parts that may not necessarily be worn out. AI-driven predictive maintenance ensures that only necessary maintenance actions are taken, which helps optimize repair schedules and extends the life of equipment. This reduces the overall cost of maintenance, including labor, parts, and machine downtime.
- Improved Equipment Lifespan By catching potential issues early, predictive maintenance helps extend the lifespan of machines and components. Replacing or repairing parts before they fail can prevent further damage to the equipment, ensuring that it continues to operate at peak performance for a longer period of time. This contributes to a better return on investment (ROI) for manufacturing assets.
- Enhanced Safety Predictive maintenance can prevent catastrophic equipment failures that could pose safety risks to workers and the surrounding environment. By detecting early signs of mechanical failure, AI systems can trigger timely interventions, helping to ensure a safer working environment and minimizing the risk of accidents.
- Data-Driven Decision Making AI-driven predictive maintenance provides manufacturers with actionable insights based on data. By leveraging these insights, decision-makers can better understand equipment performance, optimize production workflows, and make informed decisions on asset management. This data-driven approach empowers manufacturers to adopt a more proactive, efficient maintenance strategy.
- Improved Resource Allocation AI-driven predictive maintenance helps prioritize maintenance tasks based on the severity of the issue and the impact on production. This allows manufacturers to allocate resources more effectively, ensuring that maintenance teams focus on the most critical problems first while minimizing unnecessary work on less urgent tasks.
Use Cases of AI-Driven Predictive Maintenance in Manufacturing
- Automated Equipment Monitoring In a manufacturing plant, AI-powered sensors can be deployed on equipment such as motors, pumps, and conveyors to monitor their performance. These sensors track parameters such as vibration, temperature, and speed, providing real-time data to the AI system. When the system detects any abnormal patterns—such as a rise in temperature or unusual vibrations—it can send an alert to the maintenance team, signaling the need for a closer inspection or intervention.
- Quality Control and Production Optimization AI-driven predictive maintenance not only predicts failures but can also help optimize the overall production process. For example, predictive models can analyze the performance of machines involved in quality control checks, ensuring that machines operate within optimal parameters. This helps maintain consistent product quality while preventing defects or waste caused by equipment malfunctions.
- Supply Chain and Inventory Management Predictive maintenance can also improve supply chain efficiency by preventing production delays due to equipment failure. By ensuring that machines are running at full capacity and predicting when replacement parts may be needed, manufacturers can better manage inventory and avoid delays in production schedules.
- Energy Efficiency AI systems can optimize the energy consumption of machinery by predicting when equipment is likely to underperform due to inefficiency or wear. By addressing these issues proactively, manufacturers can reduce energy costs and minimize the environmental impact of their operations.
Challenges in Implementing AI-Driven Predictive Maintenance
- Data Quality and Integration AI-driven predictive maintenance relies heavily on data, and the quality of the data collected from sensors and machines plays a critical role in the accuracy of predictions. Poor data quality, inconsistent sensor readings, or lack of integration between data sources can impact the effectiveness of predictive maintenance systems.
- Initial Investment While AI-driven predictive maintenance offers long-term savings, implementing such systems requires an initial investment in sensors, AI software, and training. For some manufacturers, this upfront cost can be a barrier to adoption, although the ROI typically justifies the investment in the long run.
- Skilled Workforce Implementing AI in predictive maintenance requires specialized skills in data science, machine learning, and AI technologies. Manufacturers need to invest in training their workforce or hire skilled professionals to manage and optimize these advanced systems.
Conclusion
AI-driven predictive maintenance is transforming the way manufacturers approach equipment maintenance. By using AI to analyze real-time data, predict failures, and optimize maintenance schedules, manufacturers can reduce downtime, lower costs, and improve operational efficiency. As AI technology continues to evolve, predictive maintenance systems will become even more accurate, cost-effective, and widely adopted across industries. The future of manufacturing is undeniably tied to the power of AI, and predictive maintenance stands as one of the most impactful applications of this transformative technology.