
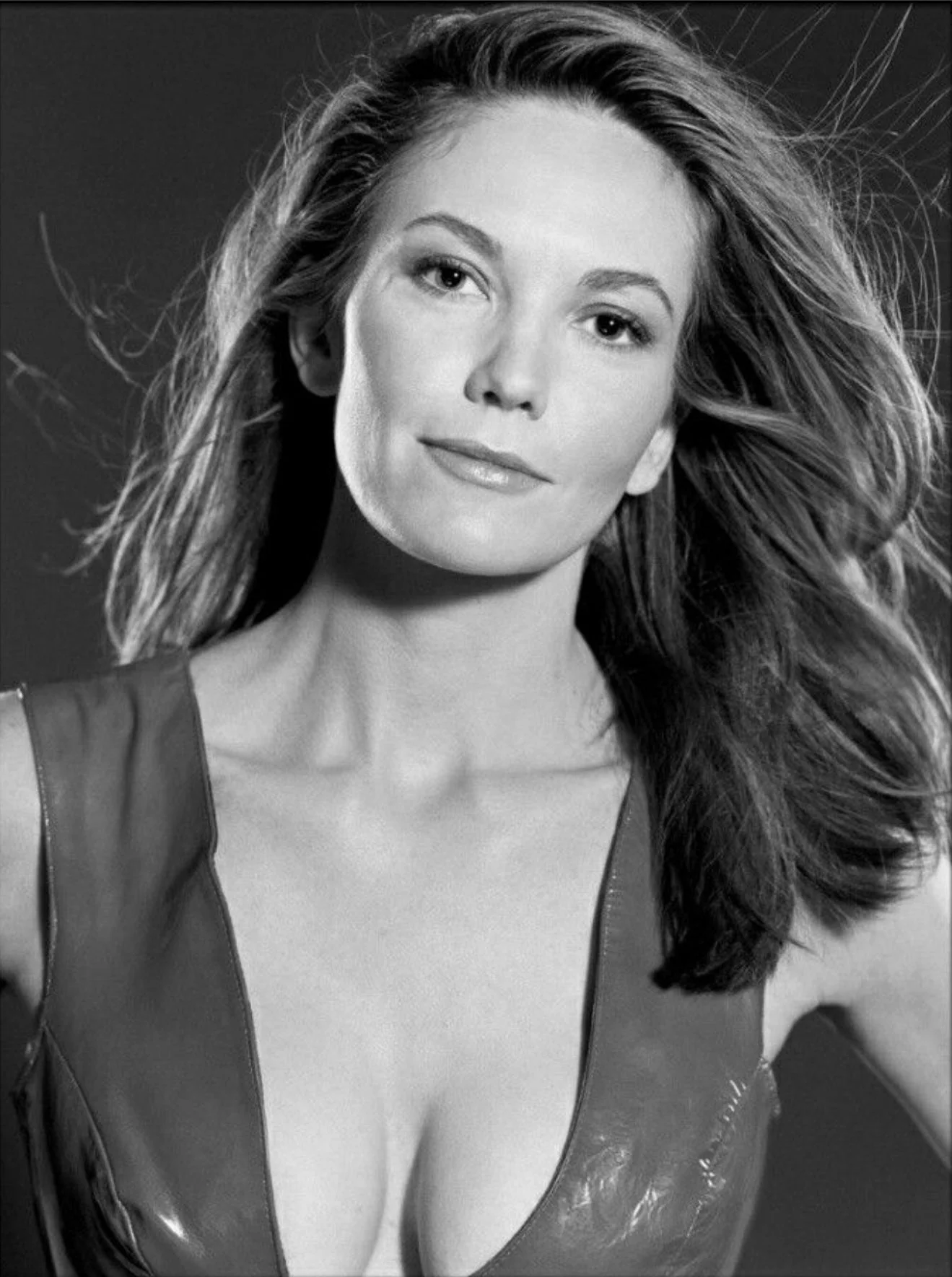

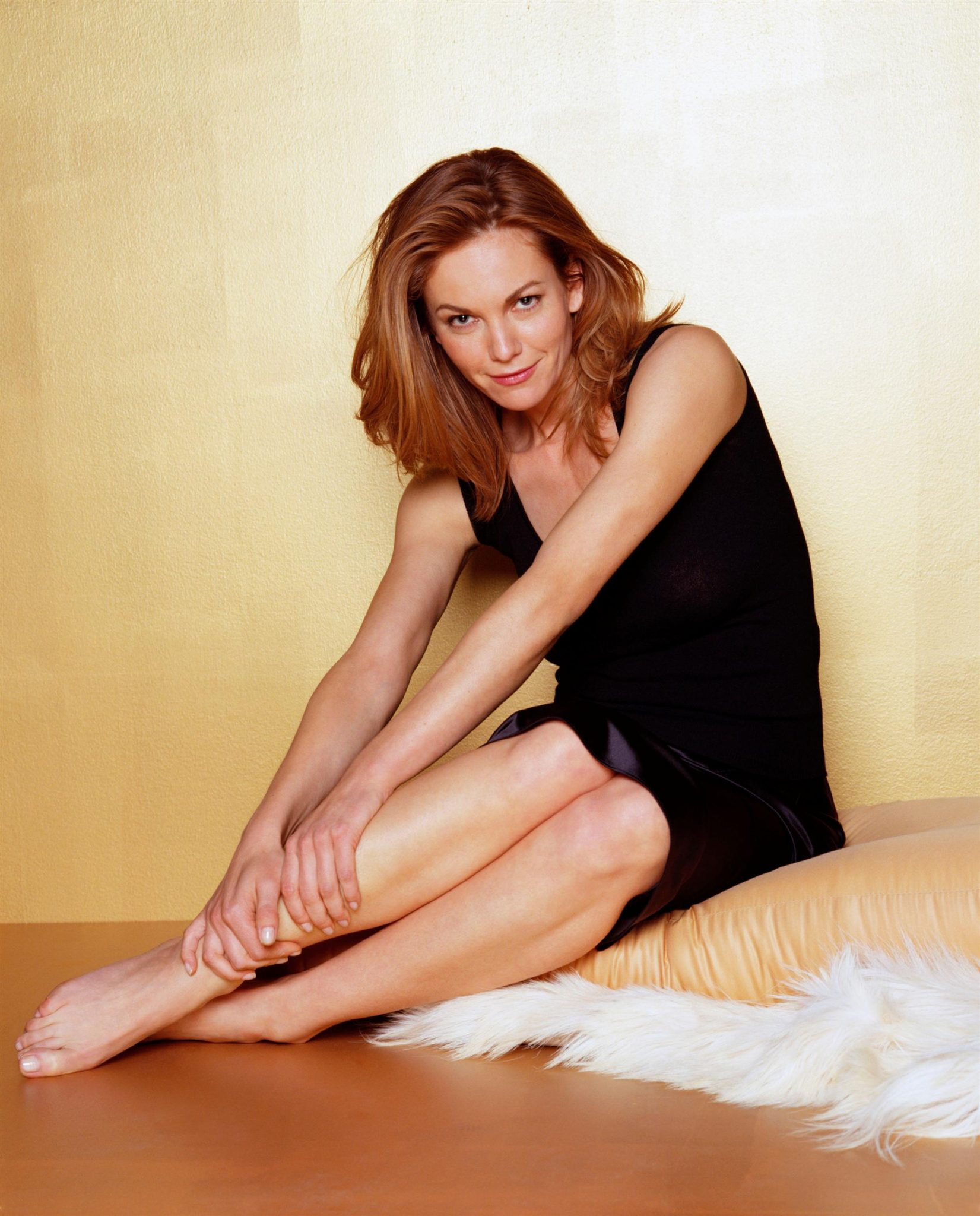
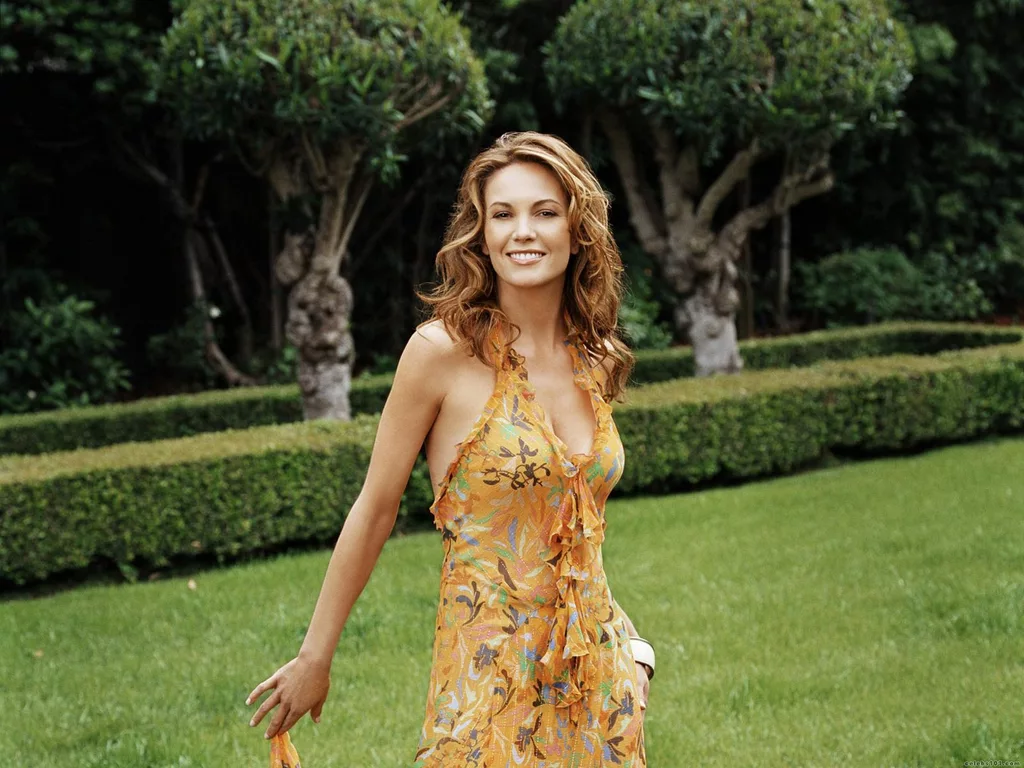
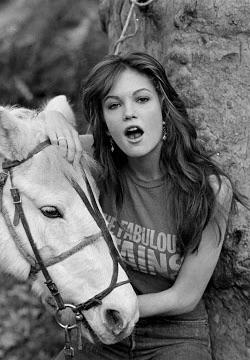
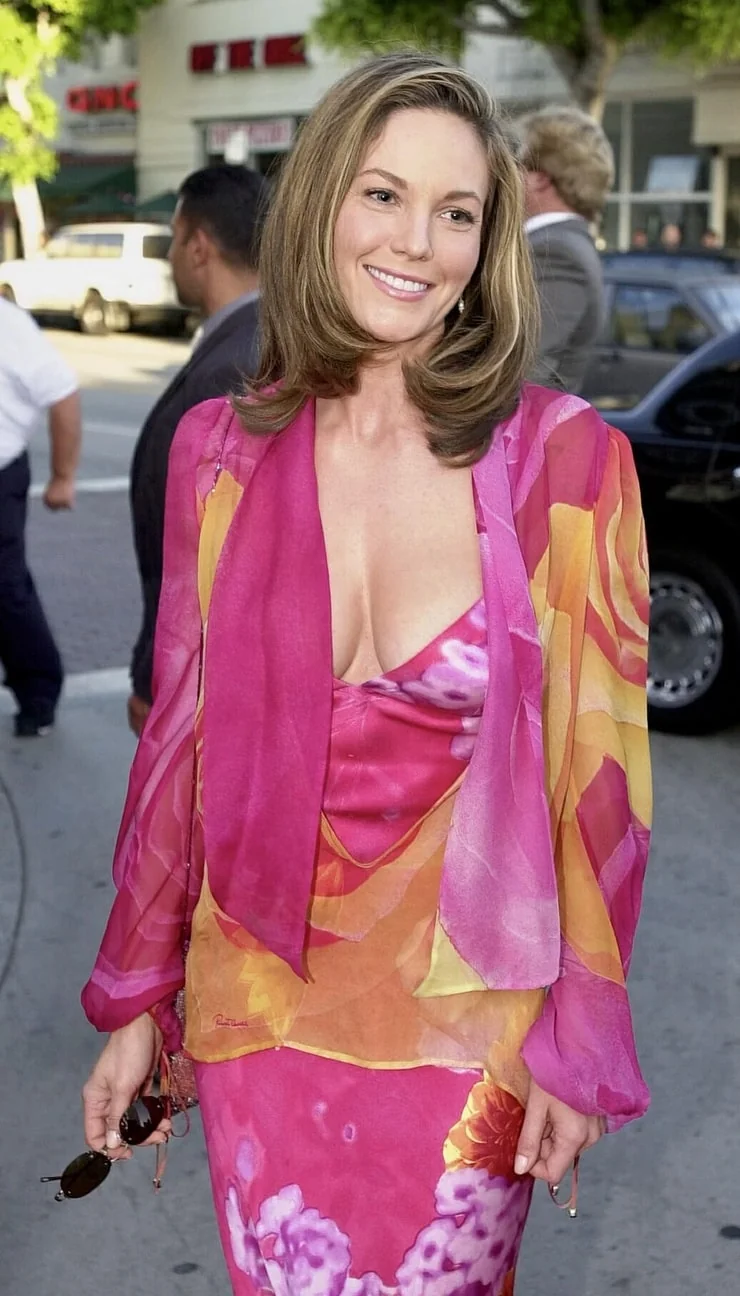
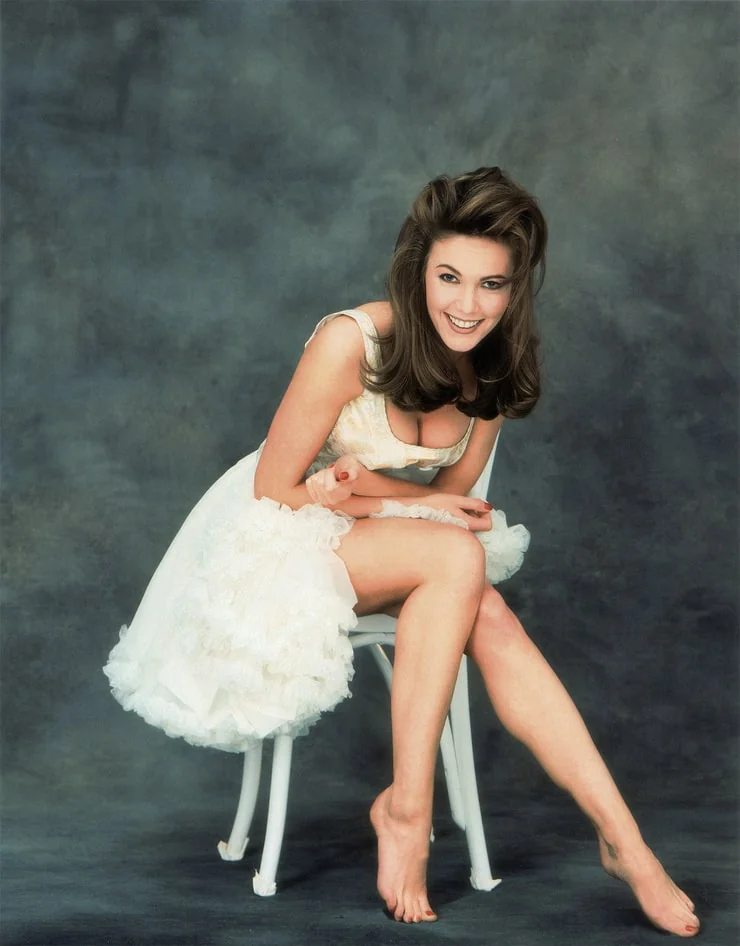
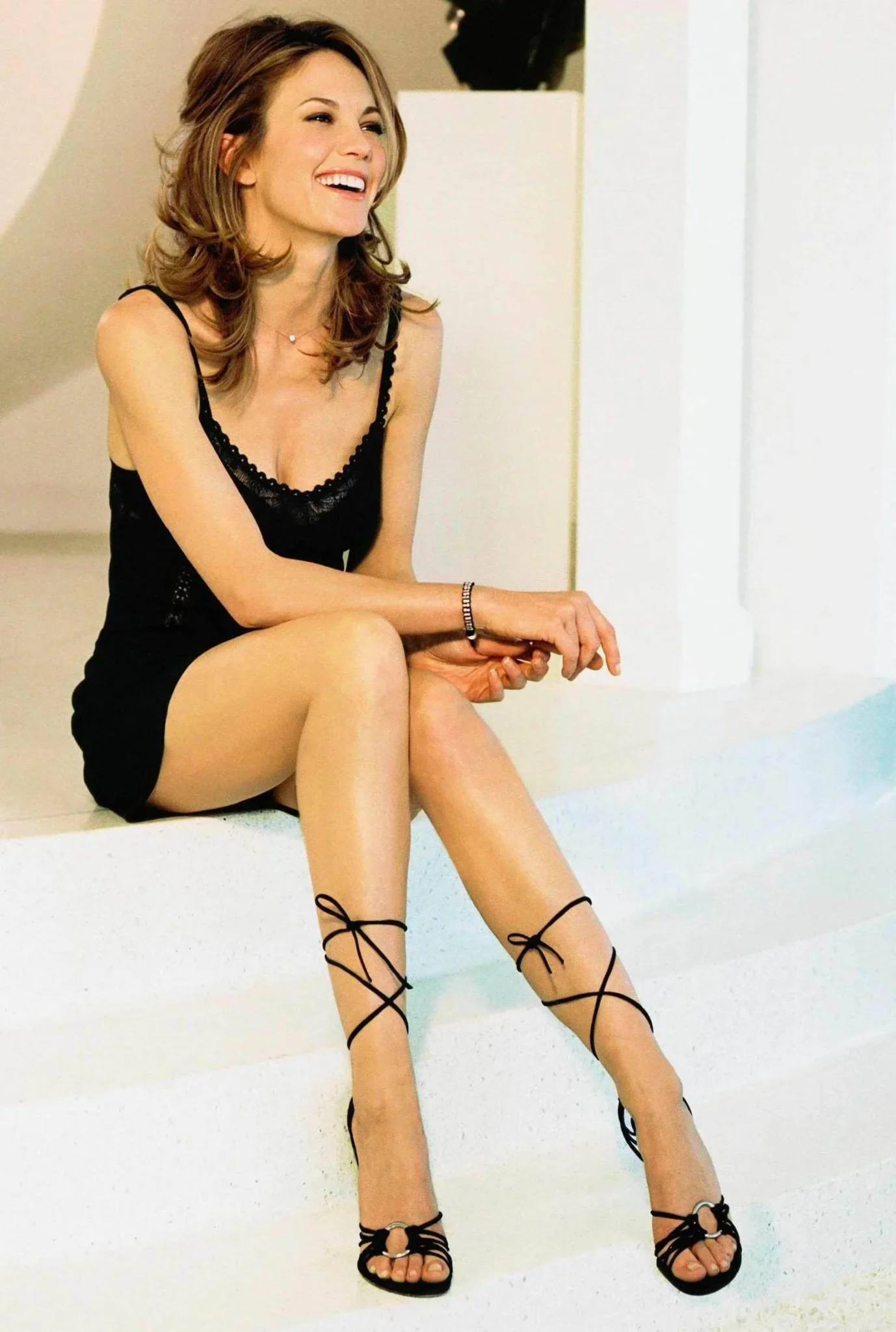
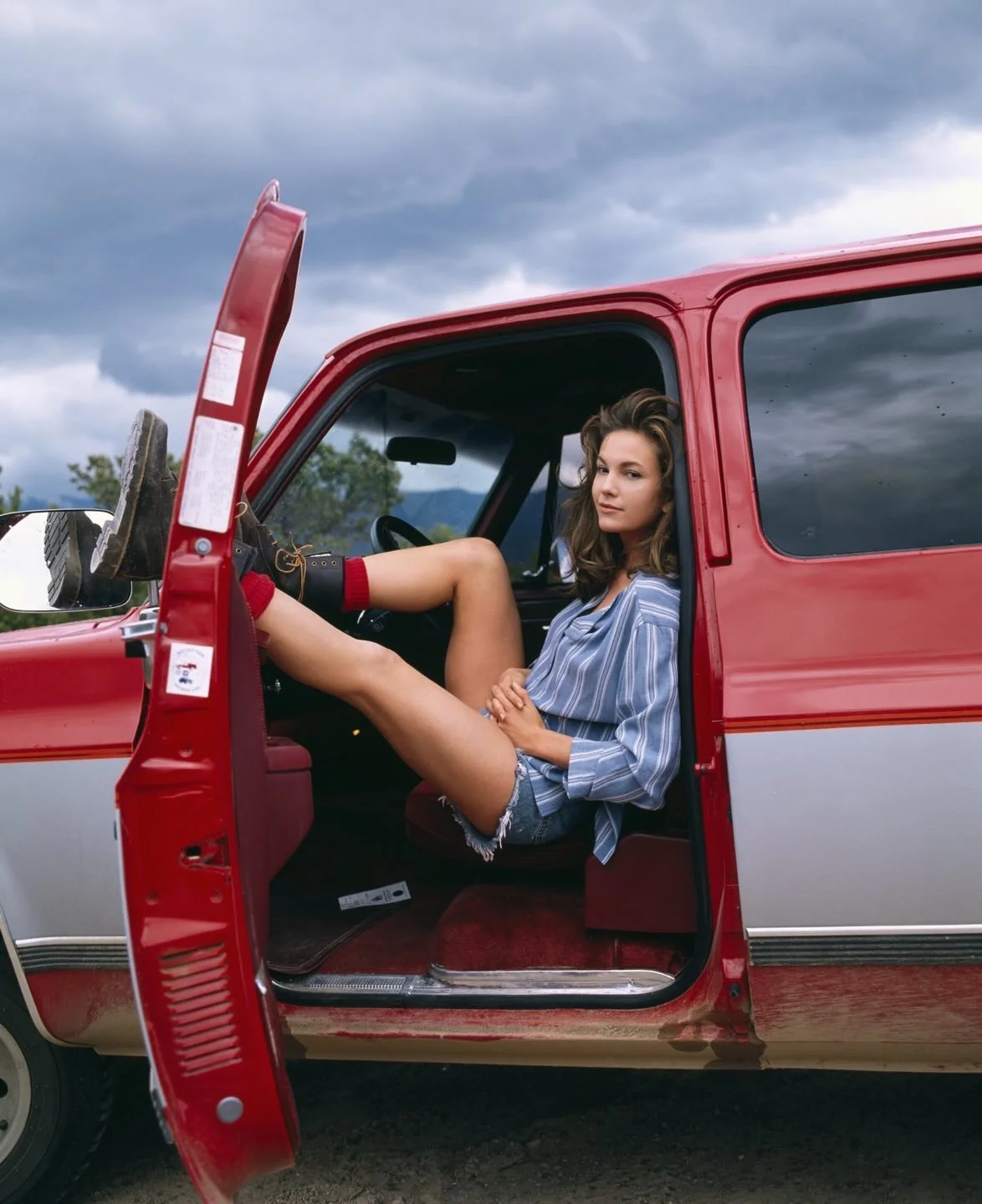
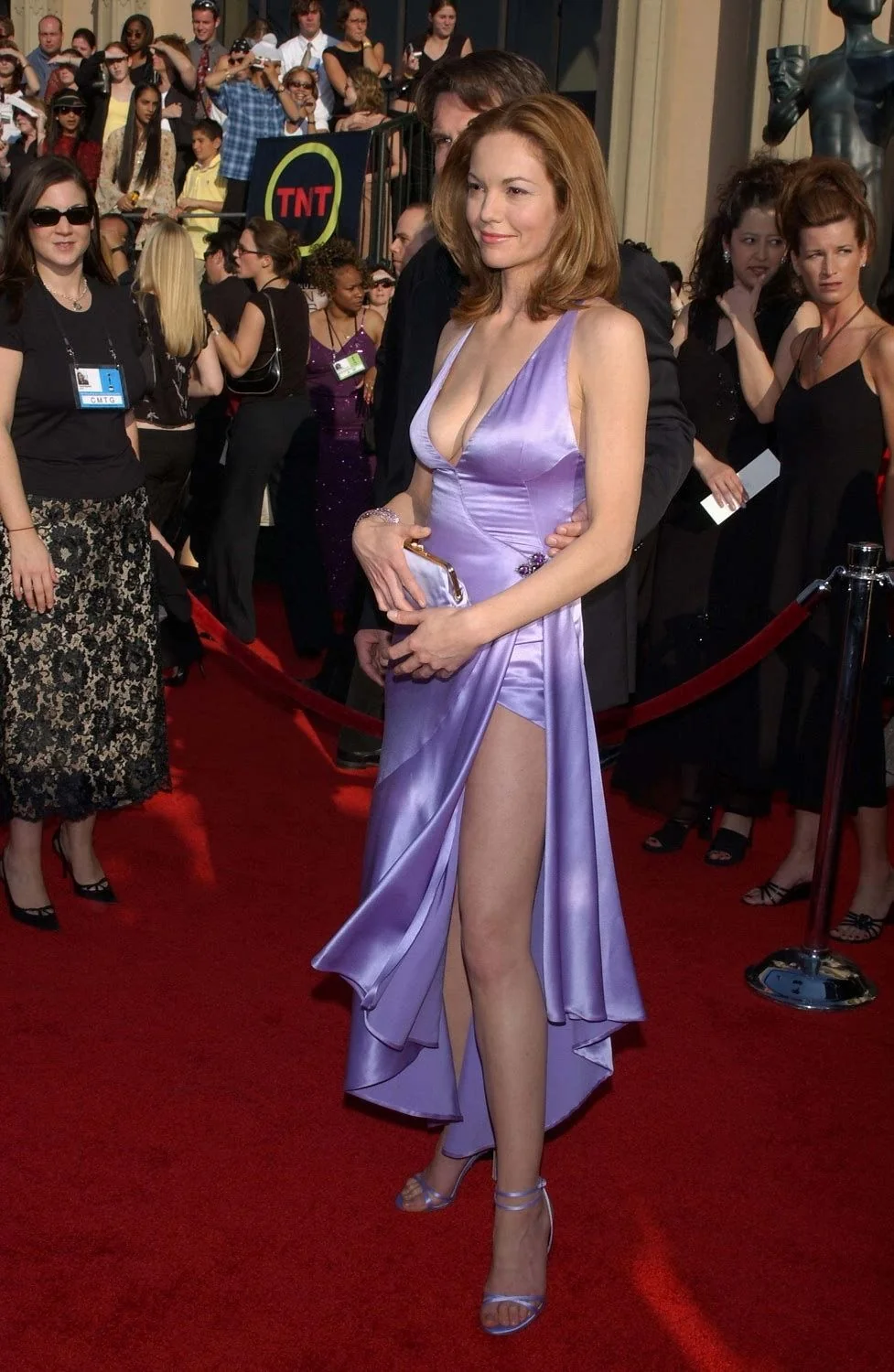
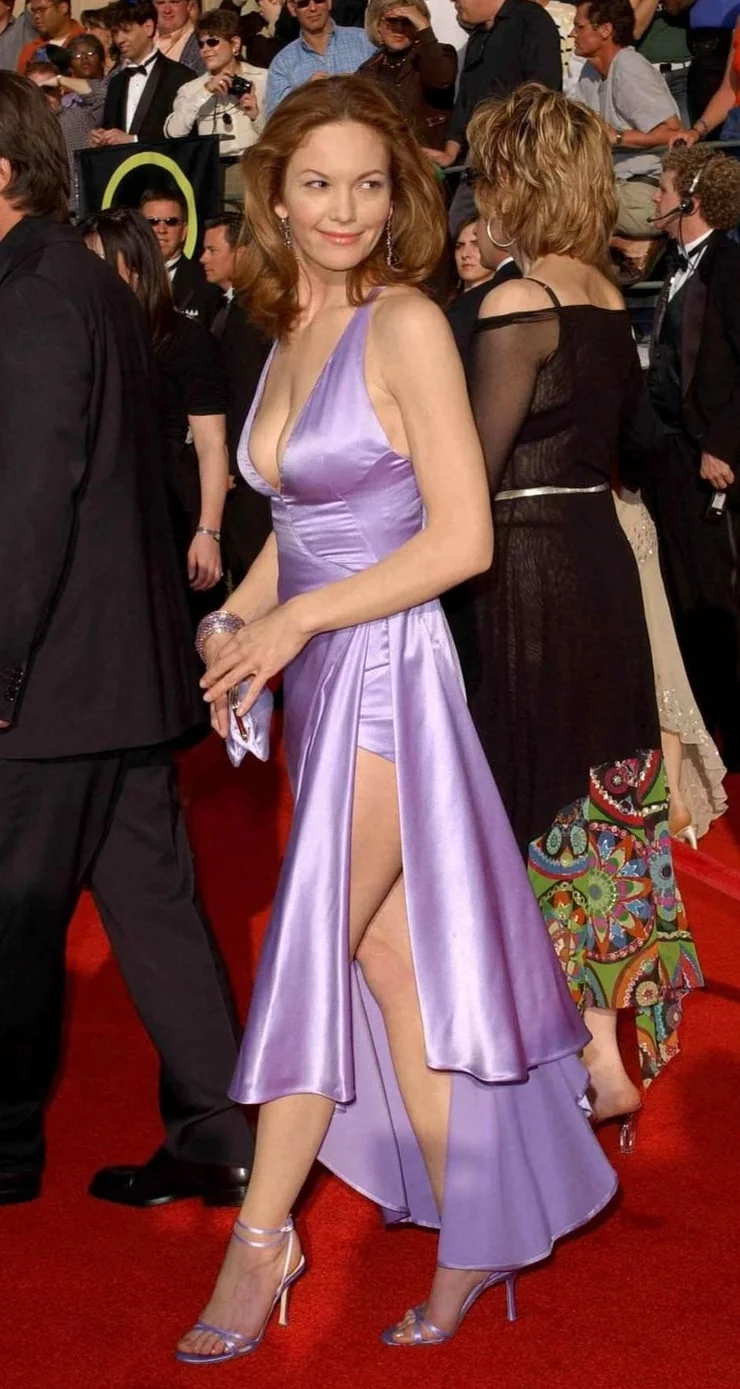
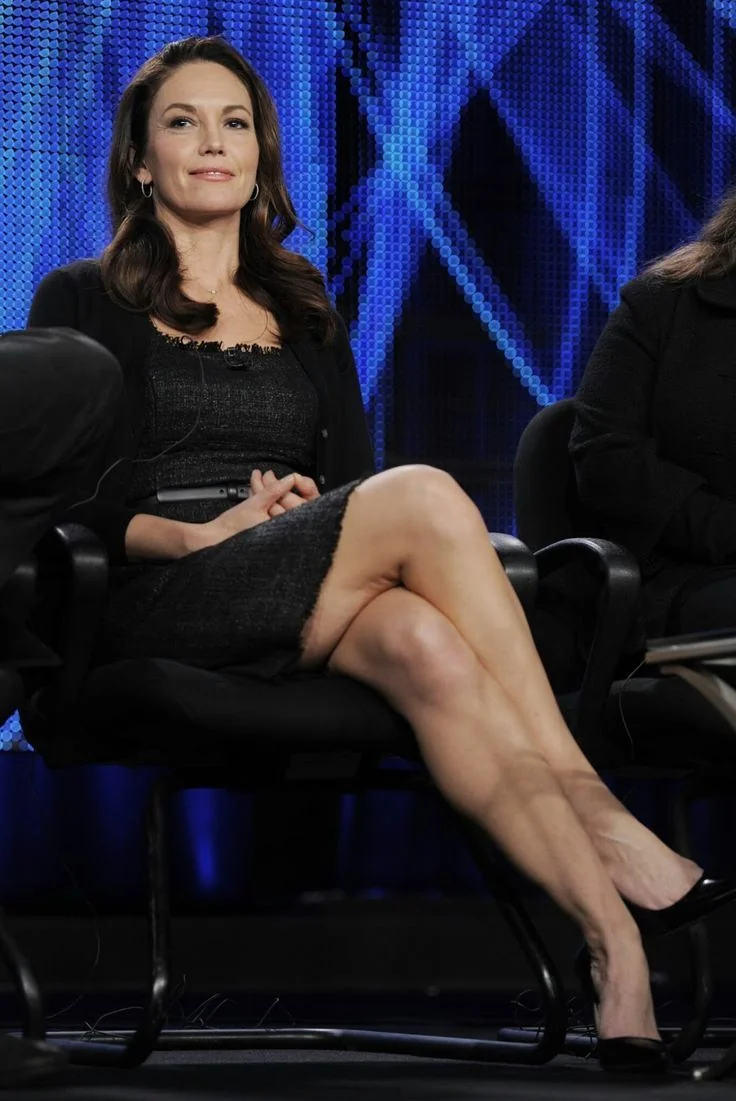

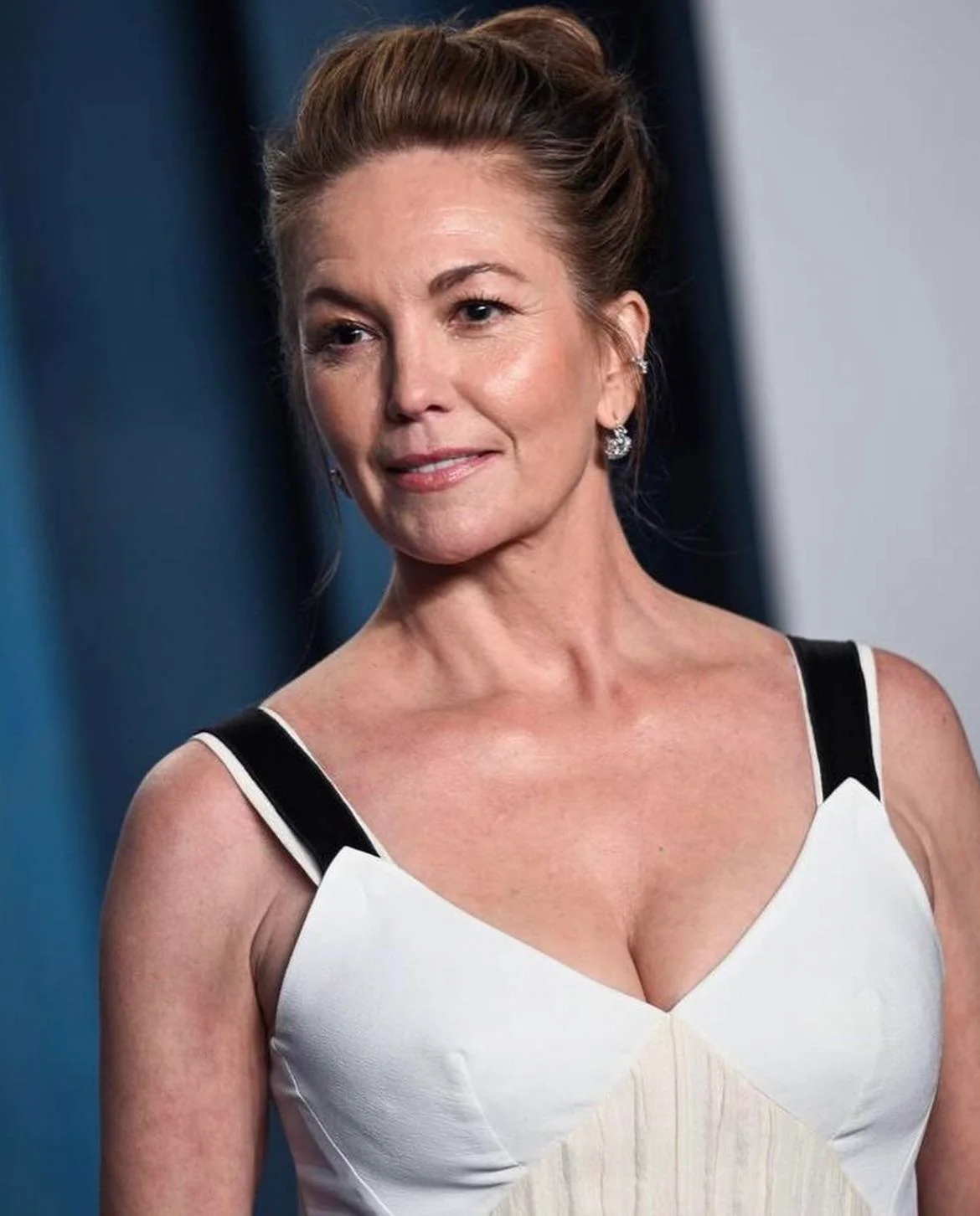
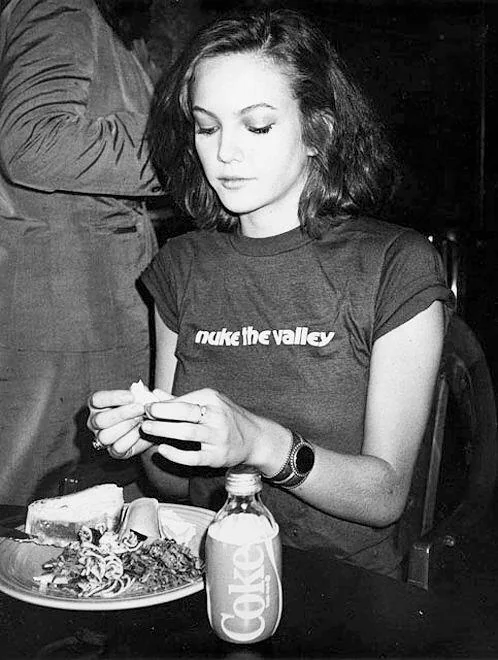
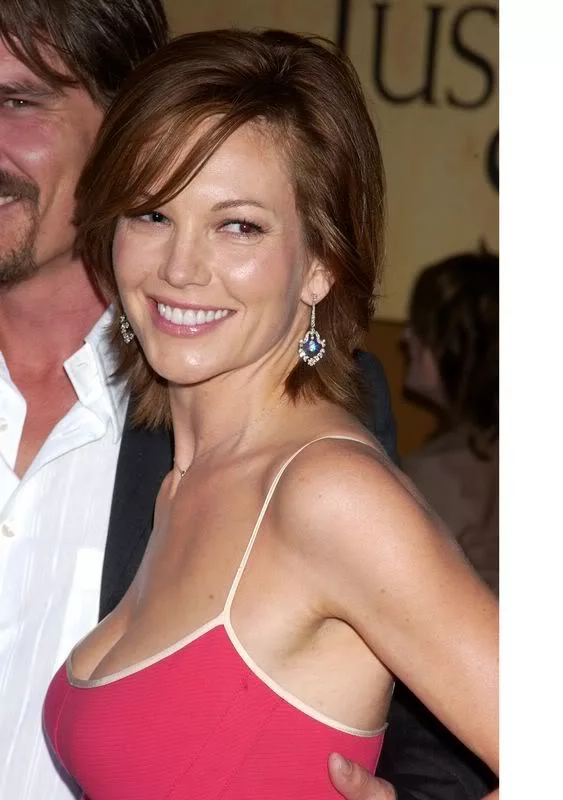
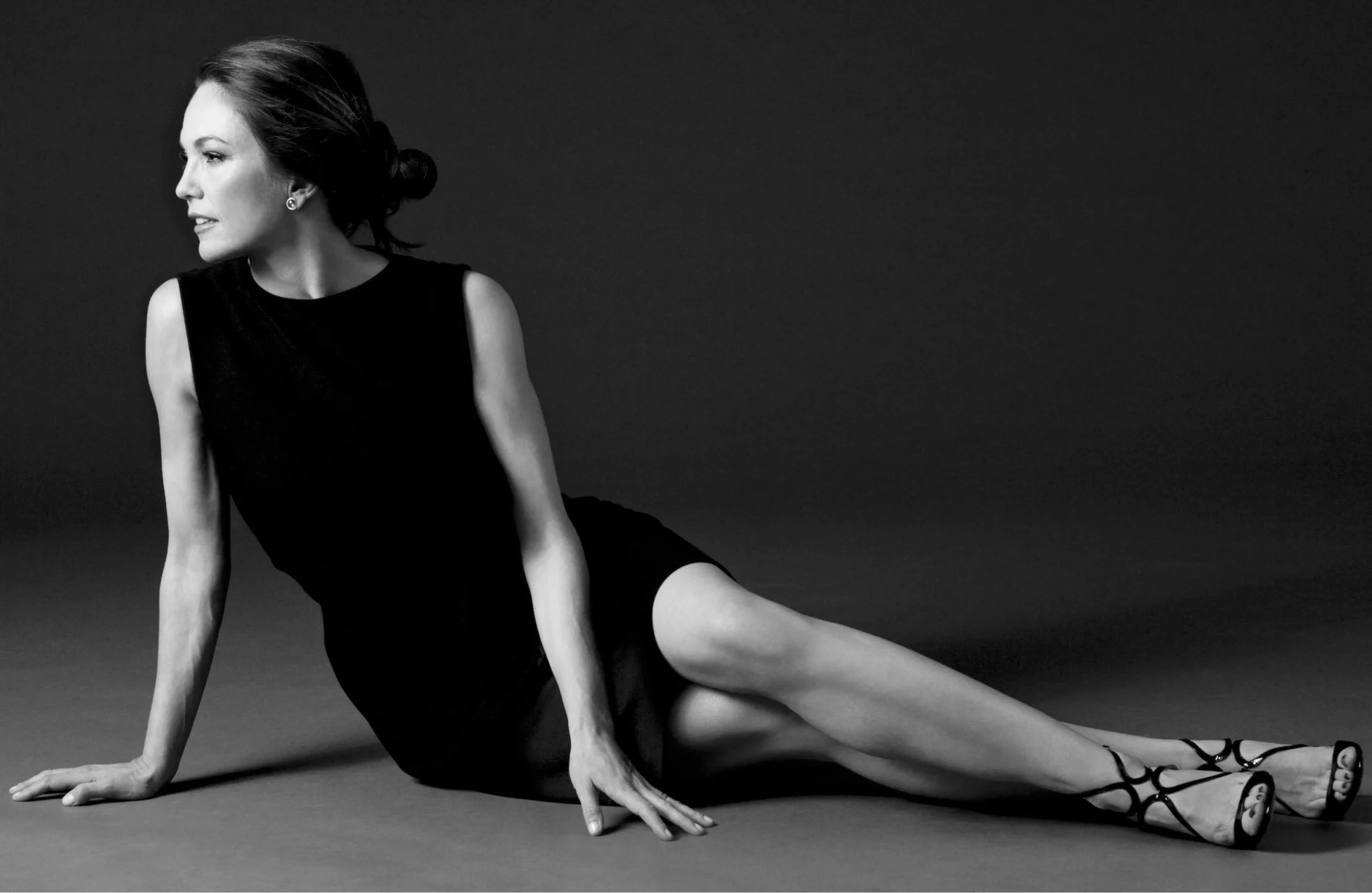
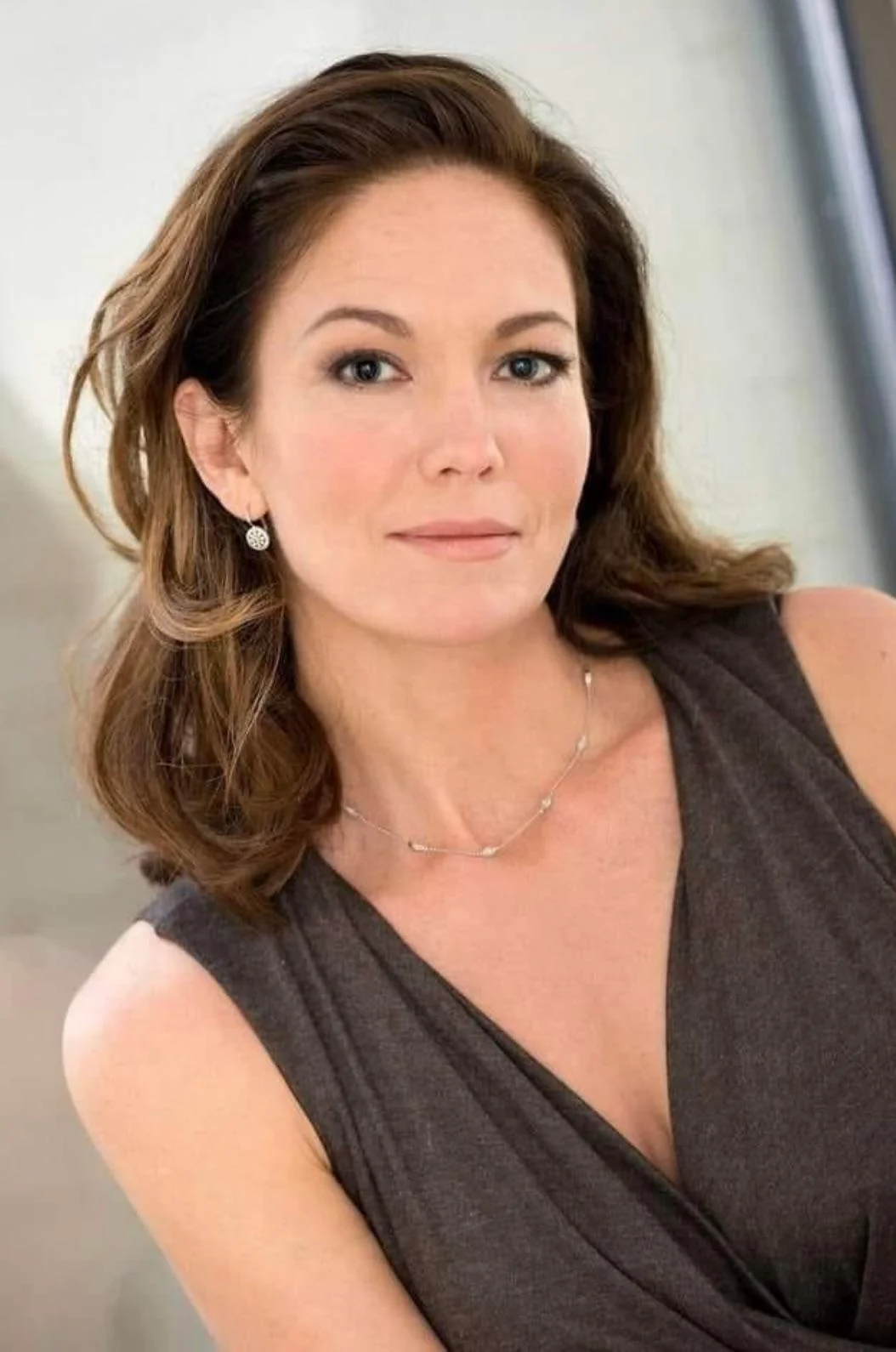

In today’s digital landscape, understanding human emotions has become more crucial than ever. With the rise of social media, online reviews, and customer feedback, businesses are inundated with data that can provide valuable insights into consumer sentiment. Enter sentiment analysis — a powerful tool that leverages natural language processing (NLP) to decode these emotions from text.
Imagine being able to gauge public opinion about your brand or product in real-time. Or pinpointing how customers feel during their interactions with your services. That’s precisely what sentiment analysis offers: a gateway to unlocking deeper connections with audiences by interpreting their feelings and attitudes through the written word.
As we dive into this fascinating world of sentiment analysis and NLP, you’ll discover its importance in modern technology, explore compelling use cases across different industries, and learn about the best tools available for harnessing this innovative approach. Whether you’re a business owner seeking competitive advantage or simply curious about how machines understand emotion-driven communication, there’s plenty to uncover here. Let’s embark on this journey together!
Understanding Sentiment Analysis and NLP
Sentiment analysis is a branch of natural language processing (NLP) focused on determining the emotional tone behind words. By analyzing text data, it helps identify whether sentiments are positive, negative, or neutral.
This process involves several techniques that break down language into understandable components. Algorithms analyze patterns in user-generated content like reviews, tweets, and comments to extract meaningful insights.
NLP plays a vital role here by enabling machines to comprehend human languages at various levels. From recognizing slang to understanding context and nuances, NLP enhances sentiment analysis accuracy.
Moreover, advancements in machine learning have improved how these systems learn from vast datasets. As they evolve, they become increasingly adept at discerning subtleties in emotion expressed through writing.
Businesses can leverage this technology for real-time feedback interpretation and better decision-making strategies based on consumer sentiment trends.
The Importance of Sentiment Analysis in Modern Technology
Sentiment analysis plays a pivotal role in modern technology. It allows businesses to sift through massive amounts of data and extract valuable insights about customer opinions and emotions.
By understanding how people feel about their products or services, companies can make informed decisions that enhance user experience. This capability is essential for maintaining competitive advantage.
Moreover, sentiment analysis fuels advancements in artificial intelligence. It helps machines interpret human emotions more accurately, paving the way for improved interactions between humans and technology.
Social media platforms leverage this tool to gauge public sentiment on various issues. This information is vital for brands looking to align their strategies with audience expectations.
In industries like finance, sentiment analysis aids in predicting market trends based on consumer behavior. The power of these insights shapes marketing campaigns and product development across sectors.
Top Use Cases for Sentiment Analysis in Business
Businesses leverage sentiment analysis to gain insights into customer opinions. This approach helps brands understand how their products or services resonate with consumers.
Social media monitoring is a prominent use case. Companies track mentions and reactions across platforms, allowing them to respond swiftly to feedback, whether positive or negative.
Customer support also benefits significantly. Analyzing interactions can identify trends in dissatisfaction, enabling teams to address issues proactively and improve service quality.
Market research stands out as another vital application. Organizations analyze public sentiment toward competitors, helping refine marketing strategies and product offerings based on consumer preferences.
Additionally, brand reputation management relies heavily on sentiment analysis. By gauging public perception over time, businesses can adapt their messaging and campaigns accordingly for better engagement and loyalty.
Best Tools and Techniques for Sentiment Analysis
When it comes to sentiment analysis in NLP, the right tools can make all the difference. Python libraries like NLTK and TextBlob are popular for their simplicity and effectiveness. They allow users to quickly perform basic sentiment tasks without overwhelming complexity.
For those seeking more advanced solutions, machine learning platforms such as TensorFlow or PyTorch offer robust frameworks. These can help in building custom models tailored to specific datasets.
Another noteworthy tool is VADER (Valence Aware Dictionary and sEntiment Reasoner). It’s particularly effective for social media texts due to its ability to handle slang and emojis.
Sentiment analysis APIs from companies like Google Cloud and IBM Watson also provide powerful capabilities. They enable businesses to integrate sentiment analysis into their applications seamlessly.
Using a combination of these tools can yield comprehensive insights, allowing organizations to stay ahead in understanding consumer sentiments effectively.
Challenges and Limitations of Sentiment Analysis
Sentiment analysis faces several challenges that can impact its accuracy and effectiveness. One major hurdle is understanding the context in which language is used. A word may have a positive connotation in one scenario but could be negative in another.
Sarcasm and irony present significant obstacles as well. Machines often struggle to detect these nuances, leading to misinterpretation of sentiments expressed by users.
Additionally, sentiment analysis tools may not account for cultural differences in communication styles. What resonates positively with one group might be perceived differently elsewhere.
The variety of languages and dialects also complicates matters. Many tools are optimized for specific languages, leaving others underrepresented or inadequately analyzed.
Data quality plays a crucial role. If the input data contains noise or bias, it compromises the reliability of sentiment predictions made by NLP systems.
Future Trends and Development in Sentiment Analysis
The future of sentiment analysis in NLP is bright and evolving rapidly. Machine learning algorithms continue to improve, enabling more accurate interpretations of human emotions across various contexts.
One exciting trend is the integration of deep learning techniques. These methodologies enhance the ability to analyze complex language structures, capturing nuances that traditional methods often overlook.
Moreover, advancements in multilingual processing will break down barriers, allowing businesses to gauge sentiments across different languages and cultures effectively. This global reach can significantly impact marketing strategies and customer engagement.
Additionally, real-time sentiment analysis tools are on the rise. Companies can now monitor social media conversations as they happen, providing immediate insights into public perception or brand reputation.
As ethical considerations gain traction, future developments may also focus on responsible data use ensuring privacy while enhancing user experience through personalized interactions.
Conclusion: The Power of Sentiment Analysis in NLP
Sentiment analysis in NLP transforms the way we interpret and interact with data. Businesses harness its power to understand customer opinions, improve products, and enhance service delivery. This technology is not just a trend; it’s becoming integral to decision-making processes.
As AI continues to evolve, so will sentiment analysis techniques. Machine learning algorithms are becoming more sophisticated, enabling deeper insights into human emotions and sentiments. Companies that leverage these advancements can gain a competitive edge.
The applications of sentiment analysis are vast—from monitoring brand reputation on social media platforms to analyzing consumer feedback across various channels. Its ability to quantify feelings provides actionable intelligence that drives strategic initiatives.
Investing time and resources into understanding sentiment analysis can yield substantial benefits. By tapping into this powerful tool, businesses can foster better relationships with their customers while adapting quickly to changing market dynamics.
Embracing sentiment analysis ensures organizations remain agile within an ever-evolving landscape where public perception matters significantly. With continuous improvements in natural language processing technologies, the future looks promising for those ready to explore this fascinating field.