











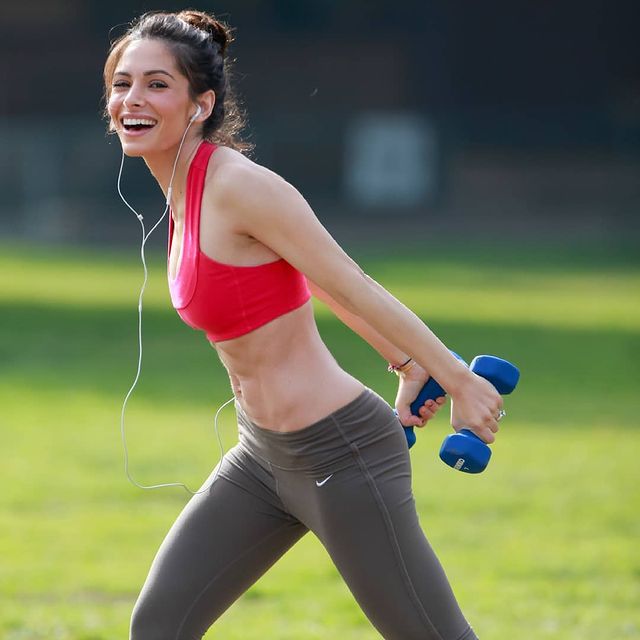




















Introduction to Data Analytics and Its Importance
In the age of information, data is more than just numbers; it’s a treasure trove of insights waiting to be uncovered. Businesses are racing to harness this valuable resource, and at the heart of their success lies data analytics. With vast amounts of data generated every day—from consumer behavior to market trends—understanding how to analyze this information effectively can make or break an organization.
Statistics in data analytics serve as the backbone for interpreting complex datasets. By transforming raw numbers into actionable insights, statistics reveal patterns that drive decision-making and strategy development. As we dive deeper into this fascinating world, you’ll discover how statistical techniques empower businesses to navigate challenges and seize opportunities like never before. Join us on this journey as we explore the best statistics in data analytics!
Role of Statistics in Data Analytics
Statistics play a crucial role in data analytics, serving as the backbone for interpreting complex datasets. By applying statistical methods, analysts can extract meaningful insights from raw information.
Descriptive statistics help summarize and describe features of a dataset. These basic techniques provide an overview that aids in understanding patterns and trends.
Inferential statistics take it a step further by allowing predictions about a population based on sample data. This is essential for making informed decisions without needing to analyze every single piece of data.
Moreover, statistical models enable businesses to identify relationships between variables. Understanding these correlations leads to better strategies and improved outcomes.
In essence, statistics empowers analysts with the tools needed to transform numbers into actionable insights, driving smarter business decisions across various industries.
Top Statistical Techniques Used in Data Analytics
Statistical techniques form the backbone of data analytics. They help analysts extract insights from complex datasets.
Descriptive statistics provide a summary through measures like mean, median, and mode. These metrics reveal trends and patterns at a glance.
Inferential statistics allow us to make predictions about populations based on sample data. Techniques such as hypothesis testing and confidence intervals are vital here.
Regression analysis is another powerful tool that establishes relationships between variables. It helps in forecasting outcomes by analyzing historical data trends.
Moreover, clustering techniques categorize similar observations into groups without prior labels. This method uncovers hidden structures within the dataset.
Time series analysis focuses on temporal data trends, making it essential for forecasting future values based on past performance.
Each technique serves a unique purpose but collectively enhances our understanding of vast amounts of information in data analytics contexts.
Case Studies: Real Life Examples of Successful Data Analytics using Statistics
Case studies highlight the transformative power of statistics in real-world data analytics. One notable example comes from Netflix, which employs statistical models to analyze viewer habits. By examining user data, they tailor content recommendations that keep subscribers engaged longer.
Another striking instance is in retail giant Walmart. The company utilizes predictive analytics to optimize inventory management. Through sophisticated statistical techniques, Walmart forecasts demand patterns based on historical sales data and seasonal trends. This not only minimizes stockouts but also reduces excess inventory costs.
The healthcare sector has seen similar success stories. Hospitals leverage statistical analysis to improve patient outcomes by identifying risk factors for diseases through extensive data sets. These insights enable targeted interventions that enhance overall care quality.
Each case underscores how robust statistics can drive strategic decisions across various industries, leading to growth and efficiency while showcasing the versatility of data analytics.
Advantages of Using Statistics in Data Analytics
Utilizing statistics in data analytics offers a plethora of advantages. First and foremost, it enhances decision-making processes. By interpreting data through statistical methods, organizations can identify trends and patterns that inform strategic choices.
Moreover, statistics provide quantifiable evidence for hypotheses. This objectivity helps teams validate their assumptions with concrete numbers rather than gut feelings.
Another benefit is predictive analysis. Statistical models can forecast future outcomes based on historical data, allowing businesses to anticipate market shifts or customer behaviors effectively.
Additionally, using statistics fosters better communication among stakeholders. Visual representations of statistical findings make complex information more digestible and engaging for all parties involved.
Leveraging statistical techniques increases operational efficiency. Organizations streamline their processes by pinpointing areas needing improvement through rigorous analysis of performance metrics.
Common Challenges and Limitations of Using Statistics in Data Analytics
Using statistics in data analytics is not without its hurdles. One significant challenge is data quality. If the underlying data is inaccurate or incomplete, any statistical analysis will yield misleading results.
Complexity often arises when dealing with large datasets. Analysts may struggle to apply the correct statistical methods, leading to errors in interpretation.
Another limitation lies in the assumptions that accompany many statistical techniques. These assumptions can sometimes be unrealistic, which skews findings and hampers decision-making processes.
Additionally, there’s a risk of overfitting models based on historical data patterns that do not hold true in future scenarios. This can create an illusion of accuracy while masking underlying trends.
Communicating statistical insights effectively remains a hurdle for many analysts. Misinterpretation by stakeholders can lead to poor business decisions despite solid analytical work being performed behind the scenes.
Future Trends and Innovations in Statistical Analysis for Data Analytics
The landscape of statistical analysis in data analytics is evolving rapidly. Machine learning and artificial intelligence are gaining traction, enabling sophisticated predictive models that adapt over time. These technologies enhance the accuracy of forecasts and insights derived from vast datasets.
Another trend shaping the future is the increasing emphasis on real-time analytics. Businesses now demand immediate insights to respond swiftly to market changes. This shift necessitates advanced statistical tools capable of processing live data streams.
Moreover, democratization of data science is underway. More professionals, regardless of their technical background, are accessing user-friendly statistical software for analysis. This accessibility fosters a culture where insights can be drawn by anyone within an organization.
Ethical considerations in statistics are becoming paramount as well. As organizations harness more personal data, transparency and responsible use will guide innovations in this field moving forward.
Conclusion
Data analytics has emerged as a vital component in the decision-making processes of businesses across various sectors. The integration of statistics enhances the accuracy and reliability of insights drawn from complex data sets. With the right statistical techniques, organizations can unlock patterns and trends that drive strategic initiatives.
Statistics play an essential role in transforming raw data into meaningful information. By employing methods such as regression analysis, hypothesis testing, and time-series analysis, analysts can derive actionable insights from vast amounts of data. This analytical power allows companies to predict future market trends, understand customer behavior, and optimize operations effectively.
Real-life examples underscore the significance of statistics in data analytics. Companies like Netflix utilize advanced statistical models to recommend content tailored to individual preferences based on viewing history. Similarly, Amazon employs predictive analytics to manage inventory efficiently by analyzing purchasing behaviors through sophisticated statistical tools.
There are numerous advantages associated with using statistics in data analytics. Enhanced decision-making capabilities arise from accurate predictions and informed strategies backed by robust analyses. Organizations often experience improved operational efficiency as they identify areas for cost reduction or performance enhancement through careful examination of their metrics.
However, it’s important to acknowledge common challenges encountered when applying statistics within data analytics frameworks. Issues such as misinterpretation of results due to bias or inadequate sample sizes can skew outcomes significantly if not approached carefully. Moreover, relying solely on historical data may lead firms astray if unexpected changes occur in market dynamics.
As we look toward the future, innovations in statistical methodologies continue reshaping how businesses approach data analysis. Advancements like artificial intelligence and machine learning will likely enhance traditional statistical techniques further—allowing for more nuanced interpretations that adapt dynamically over time.
Embracing these developments will position organizations at the forefront of industry transformation driven by insightful decisions anchored firmly in solid statistical foundations.